Smartphone-based analysis system for pancreatic islet mass quantification
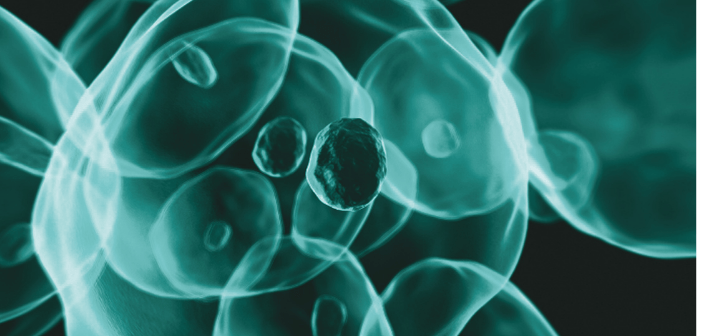
This recently published study outlines the development of a smartphone-fluidic digital imaging analysis system for pancreatic islet mass quantification. We interviewed the authors of the study to get insights on the background and significance of this new analysis system.
Many thanks for sharing insights about your work with our audience; would you please introduce yourself?
We really appreciate the opportunity to share our study with the community. My name is Yuan Xing, and I am a junior faculty in Dr Jose Oberholzer and Dr Yong Wang’s lab at the University of Virginia (VA, US). I have a broad background in biology and bioengineering, with specific training and expertise in microfluidic design, computational simulation, molecular biology and islet physiology. My research focuses on designing and developing microfluidic devices to solve biomedical problems. Dr Jose Oberholzer is a well established transplant surgeon and scientist with 20+ years of experience in islet transplant and has completed four Phase I/II and III clinical trials of islet transplant. Dr Yong Wang has extensive experience in islet physiology and microfluidics. The first author of this article, Xiaoyu Yu, is currently a graduate student in biomedical engineering. He has a strong electrical engineering background and has been trying to utilize his knowledge of electrical engineering machine learning, artificial intelligence on designing microsystems for not only the on-bench studies but also for potential clinical applications. Overall, our team is focusing on the development of microfluidic-based systems for biomedical applications, especially for diabetes research.
What is the background of your work and what were the existing challenges that you aimed to address?
Dr Xing: Islet transplants have become a successful therapy for T1D, which is able to achieve tight glycemic control without insulin injection. Defined as a ‘Biological Drug’ by the Food and Drug Administration (FDA), islet biologics for therapeutic application must meet product release criteria such as purity, viability, mass and functionality. Traditionally, islet mass quantification is often performed manually under light microscopy, which can only provide limited information (e.g. islet purity, morphology and volume), and requires operator intervention that causes significant errors and biases.
The published work aims to develop a portable, easy to use system to do islet mass assessment for the preparation of islet transplantation. We chose to use a smartphone-based digital imaging analysis (DIA) system, due to its better consistency compared to manual assessment methods. Previous DIA systems for cell related analysis often struggled in dealing with overlapping cells, and this is one of the main tasks that this work attempted to solve with the integration of microfluidic technology.
How challenging was the work on the development and validation of the new analysis system? Were there some steps that proved to be especially difficult or unexpectedly easy?
Dr Xing: One of the challenges we faced during system development and validation was the amount of data that we could get access to. It was restricted due to the shortage of human pancreas that we were offered. More specifically, such limitations made it especially difficult for the development of the algorithm for the supervised machine learning model at the current stage, so our choices of model selection are limited. The models that we have tested include k-mean-based model and the watershed-based model. Due to the variation on islets’ morphology and size, modification and tuning to all evaluated hyperparameters needed to be done every time new data was collected at the early stage of model development. This was a very time consuming procedure since the tuning needed to be made based on every case of misclassification while making sure such tuning would not affect the model’s general performance. After a long time of fine tuning and observation on counting accuracy and computational cost of two models, we eventually decided that the watershed model was the best choice for the current stage.
What are the strengths of the newly developed analysis system? Are there any downsides of using it? How does it compare to other existing analysis methods?
Dr Xing: The main advantages of our newly developed system are that it can provide a consistent assessment of the islets’ mass. At the meantime, the system can generate quantitative evaluation on islets’ parameters, such as fragmentation and purity, which are hard to be quantified by conventional manual assessments. Some of the downsides of the system include that the system requires an external pump to provide consistent flow and ensure high quality videos. Also, the assessment of volume in this paper is a 2D estimation method. The accuracy of such assessment can be improved by replacing the 2D method with 3D scanning and modeling.
What are your further work directions?
Dr Xing: Future work of the system includes improvement of both hardware and software. For the hardware, we are incorporating a pumpless microfluidic device to simplify system setup and experimental procedure. For the software, besides replacing 2D volume assessment with a 3D technique, the cloud service could be added for better computation efficiency, as well as easy data storage and access.
Furthermore, we are also upgrading our system with a fluorescent imaging module to evaluate islet cellular function by real-time live-cell dynamic monitoring. Through such an upgrade, our system will serve better as a convenient tool for, not only the physicians in preparation of islet biologics but also for researchers in their on-bench islet cell physiology studies.
Considering the overall recent scientific and technological advances, what are your forecasts for future developments and applications in this area?
Dr Xing: With the concept of ‘big data’ becoming popular in recent years, more and more clinical data will be available in this field. This makes the application of machine learning/deep learning techniques more feasible, and thus more sophisticated and accurate analysis models can be developed.
For our readers who are interested to learn more about the discussed topics – would you please recommend some valuable resources for obtaining further information?
Dr Xing: Our team has been focusing on the development of a microfluidic system for islet studies. Below are some of the related literature:
- Y Xing, P Zhang, Y He et al. Microfluidic applications on pancreatic islets and β-cells study for human islet transplant. In X Li & Y Zhou (Eds.), Microfluidic Devices for Biomedical Applications (2nd Edition). Woodhead Publishing. 617−658 (2021).
- M Nourmohammadzadeh, Y Xing, JW Lee et al. A microfluidic array for real-time live-cell imaging of human and rodent pancreatic islets. Lab Chip. 16:1466−1472 (2016).
- Y Xing, M Nourmohammadzadeh, JE Mendoza-Elias et al.. A pumpless microfluidic device driven by surface tension for pancreatic islet analysis. Biomed Microdevices. 18:80 (2016).
- Lo JF, Wang Y, Blake A, Yu G et al. Islet preconditioning via multimodal microfluidic modulation of intermittent hypoxia. Anal Chem. 85:11240−11249 (2013).
- Lee D, Wang Y, Mendoza-Elias JE et al. Dual microfluidic perifusion networks for concurrent islet perifusion and optical imaging. Biomed Microdevices. 14:7−16 (2012).
- AF Adewola, D Lee, T Harvat et al. Microfluidic perifusion and imaging device for multi-parametric islet function assessment. Biomed Microdevices. 12:409−417 (2010).
- Wang Y, Lo JF, Mendoza-Elias JE et al. Application of microfluidic technology to pancreatic islet research: first decade of endeavor. Bioanalysis, 2:1729−1744 (2010).
- Mohammed JS, Wang Y, Harvat TA, Oberholzer J, Eddington DT. Microfluidic device for multimodal characterization of pancreatic islets. Lab Chip. 9:97−106 (2009).
On the other hand, we have also done lots of studies related to Type I diabetes and islet β-cells. Below are some pieces of literature related to such topics:
- Y Wang, M Qi, JJ McGarrigle et al. Diazoxide preconditioning of nonhuman primate pancreas improves islet isolation outcomes by mitochondrial protection. Pancreas, 49:706−713 (2020).
- MA Bochenek, O Veiseh, AJ Vegas et al. Alginate encapsulation as long-term immune protection of allogeneic pancreatic islet cells transplanted into the omental bursa of macaques. Nat Biomed Eng. 2:810–821 (2018).
- Y Wang, KK Danielson, A Ropski et al. Systematic analysis of donor and isolation factor’s impact on human islet yield and size distribution. Cell Transplant. 22:2323−2333 (2013).
Finally, the following literature/resource turned out to be very helpful when developing the digital image analysis system in this work:
- AS Chowdhury, R Chatterjee, M Ghosh, N Ray. Cell tracking in video microscopy using bipartite graph matching. The 20th International Conference on Pattern Recognition. (2010).
- G Bradski. The OpenCV Library. Dobb’s Journal of Software Tools. (2000).
Interviewee: Atanas G Atanasov, research leader focusing on molecular medicine and digital health, PI of LBI-DHPS, Editor-in-Chief of CRBIOTECH, and Executive Administrator of the open innovation platforms DHPSP and INPST.