Peek behind the paper: LC–TOF–MS methods to quantify siRNAs
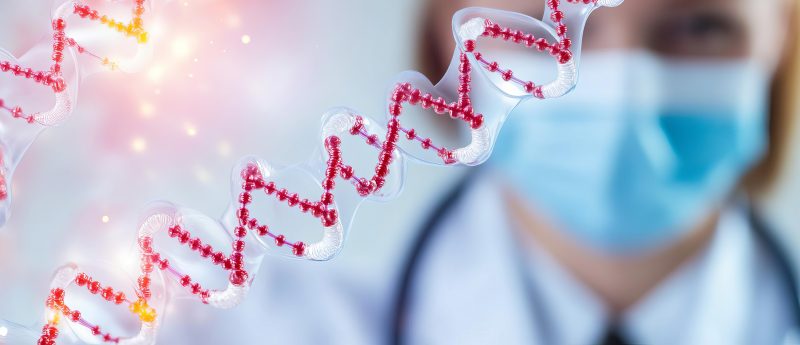
Timothy Snow joined QPS, LLC (FL, USA) in 2016 and is Senior Principal Investigator in QPS’s Metabolism group at QPS-DMPK. He is primarily involved in metabolite identification and quantitation of therapeutic oligonucleotides. Tim began his career in the oil industry developing GC/MS/MS methods for petroleum biomarkers (Conoco, Ponca City, OK, 1981). He moved on to small molecule metabolite identification and quantitation using UPLC/HRMS at DuPont’s Haskell Laboratory for Toxicology (Newark, DE, 1998) where he transitioned to larger molecule applications, principally proteomics, in support of DuPont’s systems biology effort. Tim received his BS and MS in analytical chemistry from the State University of New York at Oswego (NY, USA) and his PhD in analytical chemistry (mass spectrometry) from the University of Oklahoma (OK, USA).
A short summary of the paper ‘LC–TOF–MS Methods to Quantify siRNAs and Major Metabolites in Plasma, Urine and Tissues’ Helen Shen et al, published in Bioanalysis.
Different bioanalytical approaches have been used for the detection and quantification of therapeutic oligonucleotides, such as small interfering RNA’s (siRNA) and their metabolites. This paper specifically discusses the use of UPLC/HRAM–MS (ultrahigh pressure liquid chromatography/high-resolution accurate mass-MS) in our laboratory for validated quantitative analyses for this new class of drug. Although the molecular weights of these analytes are very high, we met the same acceptance criteria as are expected for small-molecule assays. This approach provides quantitative information for targeted oligonucleotides along with related metabolites. HRMS in full-scan mode provides selectivity and can significantly improve signal-to-noise ratios and ultimately quantitation limits. We are able to achieve a lower limit of quantitation of 5 ng/mL for the parent drug and at least that level of detection for the identification of all major metabolites. The method was successfully validated with respect to linearity, sensitivity, accuracy, precision, selectivity and recovery. Our method, which only required 100 μL of sample, was successfully used for regulated preclinical and clinical studies over 3 orders of linear dynamic range.
1. What types of therapeutic oligonucleotides have you analyzed?
Therapeutic siRNA’s are a new class of nucleic acid-based drug that can selectively silence gene expression. Typically containing a single strand RNA (antisense), ~15−30 base-pairs long, which modify or interfere with a targeted section of a DNA strand. These can also contain a second (sense) strand which forms a duplex with the functional antisense strand. This second strand acts to ‘guide’ the duplex to the site of action of the gene. Both strands can be modified to improve stability and efficacy of the formulation. For example, most therapeutic oligonucleotides are designed with phosphorothioate linkages in the backbone to improve their stability. The sense strand is also often modified with large hydrophilic end groups, such as cholesterol, fatty acids, polyethyleneglycol (pegylated) or N‑acetylgalactosamine (GalNAc) conjugates, which allow these relatively bulky duplexes to more easily penetrate cellular membranes and properly align themselves at the site of action. Degradation or metabolism of the antisense or sense strand can create side effects and/or changes in the drug’s overall efficacy, which makes their identification and quantitation extremely important for regulatory approval.
2. What is the advantage of using UPLC/(HRAM-MS) high resolution accurate mass MS for quantitative and qualitative oligonucleotide analysis?
We have found ion-pairing reversed-phase UPLC the best separation method for both quantitative and qualitative analyses of oligonucleotides. In our experience, it gives very sharp well-resolved chromatographic peaks without compromising mass spectrometric performance.
HRAM-MS has a mass selectivity advantage, which allows differentiation of individual 13C isotopes within a molecular ion cluster. Our quantitation software (MultiQuant, Sciex, Concorde, ON) automatically integrates each isotopic peak individually and excludes areas between the peaks, which are mostly electronic and chemical noise. This noise exclusion significantly improves signal-to-noise relative to quantitation acquired at lower mass resolution since with unit mass resolution the isotopic envelope is not resolved, resulting in one broad molecular ion peak, which can only be integrated to include all signal, both ‘real’ and noise-based. Quantitation using HRAM-MS characteristically results in lower detection limits, wider dynamic ranges and much improved reproducibility.
That same selectivity is very important for metabolite identification. Even though therapeutic oligonucleotide drugs only contain one or two strands, mass spectra of samples (e.g. plasma, tissue) can be very complicated. ESI mass spectra contain several different ions for a single oligonucleotide, all the observed charge state ions, all the isotopic peaks, typically 3−15 for an oligonucleotide, depending on the chain length, and all the contributions from the other oligo-related compounds that may co-elute.
Luckily, we have processing software (PeakView, Sciex, Concorde, ON) that allows us to mathematically combine, or deconvolute, all the MS signals for all multi-charged species into a combined singly-charged molecular ion cluster. The average molecular weight for this oligonucleotide can be automatically calculated with this software from the weighted average of the mass of each isotope in the cluster and the mass spectral intensity for each isotopic peak.
The first isotopic peak in this deconvoluted molecular ion cluster is its monoisotopic mass. It represents the accurate mass of the oligonucleotide if it contained only the most naturally abundant isotopes in nature for all of its atoms. Once deconvoluted, the cluster data is ‘deisotoped’ yielding a single, monoisotopic molecular ion for each species (we use Promass HR software, Novatia, Newtown, Pa), dramatically simplifying the data. This monoisotopic mass almost unequivocally identifies an oligonucleotide’s exact empirical formula. This deconvoluted, deisotoped data is then used by ProMass to look for general differences between dosed and control samples. It can even be set to search for specific oligonucleotide xenobiotic transformations.
3. Please describe how you are getting metabolism data from full scan HRAM-MS data that was acquired specifically for quantitation.
One advantage of full scan HRAM-MS data versus multiple ion monitoring or even multiple reaction monitoring data is that all ions over a wide mass range are acquired (~900−3000 Da), instead of several narrow mass ranges. We have seen very little difference in the quality of quantitative results when acquiring wide mass windows with a time-of-flight mass spectrometer. This allows interrogation of the data for oligonucleotides or related compounds weeks or months after the original acquisition. Generally, a longer shallower LC gradient is used for metabolite identification, about 20 to 50 minutes versus 3 to 5 minutes for quantitative analysis, but we have found that short runs can still provide very good qualitative data. This also allows us to look for transient metabolites at individual time points, which can sometimes be obscured in pooled metabolite screening samples.
4. What are some of the challenges in metabolite oligonucleotide identification/quantitation? What types of metabolites have you seen?
Luckily, oligonucleotides metabolize in fairly predictive ways. Exonuclease and endonuclease cleavage is by far the most prevalent mechanism in oligonucleotide biotransformation. Generally, cleavages occur at the phosphate linkage, leaving a free phosphate group at one or both ends of the metabolic species. This cleavage usually occurs sequentially (n-1, n-2, n-3, …, n-x) with loss of one monomer at a time from the 3’ or 5’ end. Although less commonly, endonucleases can attack the middle of the oligonucleotide to yield metabolites with seemingly random chain lengths. The challenge for quantitation of this class of metabolite, and most others, is that reference standards are not readily available.
Therapeutic oligonucleotides can be designed to be less resistant to degradation and metabolism. Often an oxygen is replaced with a sulfur in some phosphate linkages (phosphorothioate groups) for better stability. A common metabolic mechanism for this group is a reversal of this exchange of the sulfur back to an oxygen. At low resolution this conversion looks like a simple loss of an oxygen, HRAM-MS is necessary to confirm this is an exchange of sulfur for oxygen, rather than a loss of oxygen, thanks to the discrete differences in accurate mass. The biological significance of this exchange is unknown but it is typically measured to determine the extent of degradation of the drug.
A final challenging application of HRAM-MS to therapeutic oligonucleotides is confirmation of oxidative deamination. This transition usually only occurs on end groups containing the base adenosine. This metabolism step results in an increase of only 1 Da on metabolite of about 8000 Da but can be easily resolved in a -3 to -5 charge state ion by HRAM-MS.
5. At QPS, what are you hoping to do next in oligonucleotide research? What do you think the future holds?
Improvements in mass spectrometer source design and faster, better resolving chromatographic options are constantly being delivered. New column chemistries for LC separation and solid phase columns for sample preparation need to be evaluated as they become available. Microflow HPLC shows great promise, performed at 1−10 μL/min rather than 800−500 μL/min, and it has been demonstrated to increase oligonucleotide MS sensitivity as much as 10-fold. MS/MS is a technique that we would like to better utilize for highly selective quantitation methods and to improve confidence structure elucidation, specifically end group analysis. Finally, hopefully soon, Artificial Intelligence (AI) advances in software development will gradually increase throughput, by making automated processing software much faster and smarter.
In association with: