Opening metabolomics’ black box
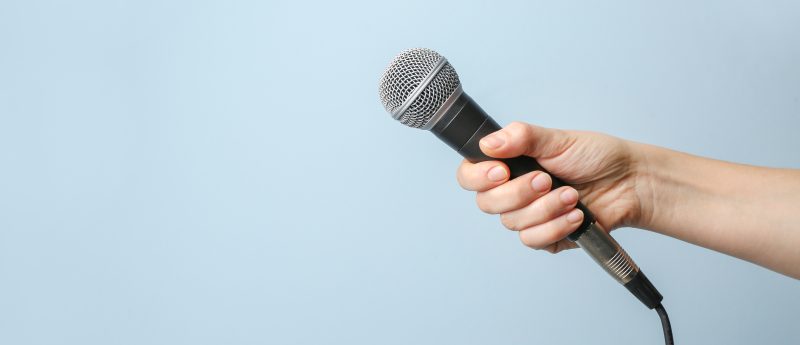
Professor Coral Barbas is currently a Full Professor of Analytical Chemistry at Pharmacy Faculty, Universidad San Pablo CEU, (Madrid, Spain) and Director for the Centre for Metabolomics and Bioanalysis (CEMBIO) at this Faculty. She is also Director for CEU International School of Doctorate (CEINDO); Visiting Professor at Imperial College London (UK), Department of Surgery and Cancer. As previous appointments she was Vice-Chancellor for Research at Universidad CEU San Pablo (Madrid, Spain) and Marie Curie Fellow at Kings College London (UK) and visiting Professor at Bialystok Medical University (Poland).
She is the author of more than 250 papers, with current research interests in all the steps in metabolomics workflow: experimental design, sample pretreatment, analytical methods for targeted and untargeted metabolomics, method validation, data treatment, compound identification and interpretation. Her researcher is focused on multiplatform analysis with GC–MS, LC–MS and CE–MS of all kind of biological samples searching for disease biomarkers, prognostic biomarkers, mechanisms of action of a drug, diet, etc.
Her awards include Angel Herrera Research Award and Teaching Award, the medal of Bialystok Medical University and she was named to the 2016 Power List, the 50 Most Influential Women in Analytical Chemistry in the World, The Analytical Scientist and recently she has received the award of the Belgian Society of Pharmaceutical Sciences (BSPS 2018) and a Honoris causa doctorate in Bialystok Medical University Member of different boards of international Committees and Editor for Journal of Pharmaceutical and Biomedical Analysis.
My research journey started with developing analytical methods for the biochemistry lab, in particular, vitamin E related compounds in every type of matrix, as at that time vitamin E – a potent antioxidant – was considered a potential cure of all kinds of diseases. Later, when I started to lead the Analytical Chemistry Area of a Pharmacy Faculty, my research evolved towards the over-demanding analytical rules of the pharmaceutical world: Development and validation of analytical methods for quality control of pharmaceutical formulations, stability studies, impurities identification and quantification. At first, discovering the capabilities of different types of chromatographic columns, the difficulties of achieving 100% recovery, relative standard deviations lower than 3% or the importance of rigorous data treatment procedures was interesting and without a doubt gave me a solid analytical background. I have benefited from it along my career, although I still think that separation sciences are also a pinch of art. However, once I had mastered all those aspects, I began to consider the incredible analytical capabilities that we had in the lab with the arrival of a mass spectrometer that could be useful for many more things and I started to be interested in the incipient metabolomics technology.
Metabolomics, aiming for the identification of ALL altered metabolic pathways in response to a pathophysiological process or voluntary intervention in a biological system, is an incredible research tool if properly used. Never before, discovery capabilities for new biochemical findings were at this level.
To compare differences between classical, hypothesis based research, and ’omics’ technologies, I use to say that if you go to catch a trout and you select the best day, the place, the light and the bait, you probably will come back home with a trout, but if you go to the high sea throw a big net and catch everything around, you could find the most unexpected things, including a mermaid! Although after a huge effort and outflow of time and money you can also end with a net full of plastic bags and rubbish… In any case you should be prepared to identify your findings and their value. If you think that the TCA cycle should be altered in your model and you measure the corresponding compounds, you can either confirm or disregard your hypothesis and in the latter case you have nothing. But if you measure every metabolite that is altered in your system, without a priori hypothesis, you can discover that instead of the TCA cycle, fatty acids are increasing and that can lead to generate a totally new hypothesis.
Coming from a strict pharmaceutical analytical background, metabolomics seemed to me that it was breaking all the classical rules, and not only to me, a reviewer wrote once in a grant application that I presented: “if you don’t know what you want to measure how you are going to measure it”. Nevertheless, I was converted to metabolomics after my first experiment. I was in the cafeteria when I overheard the conversation between two colleagues; they had an experiment with schistosomiasis infected mice and they wanted to measure a lot of compounds, however, one of them said: “but we only have one single drop of urine”. I turned around and after excusing my intromission, offered to apply capillary electrophoresis using a metabolomics approach. I developed the profiles, applied multivariate analysis to obtain a PCA plot and… there was a nice classification of most of the animals in two groups except one of the infected mice, which was clustering together with controls, what a pity! All of a sudden, someone appeared at the entrance of my office and said: “look, Coral after the autopsy, we learned that one of the mice was not infected” and I responded, “number 8”, a face full of astonishment looked at me and asked: “how do you know?” Metabolomics discovers the patterns under sample classification, a different question is if we are able to identify the strongest set of variables responsible for that classification and interpret their biological meaning.
However, currently, many researchers, blinded by the flash of new technologies, think that metabolomics is mostly a question of learning how to use the proper software tools and press the right key until you get a fancy set of plots. It is true that mastering metabolomics requires an impressive knowledge in bioinformatics, however even more importantly it requires a deep knowledge of your biological question, the samples selected to approach it and the effect that every step in both the analytical process and data treatment is producing on them.
Analytical chemists are trained to think about every step in the analytical process and that cannot be forgotten when doing metabolomics. Metabolomics is not a black box where you put samples in and get data out. Every step along the analytical process must be considered with the same detail as if it is a targeted or an untargeted measurement.
There are several recent examples in our research. We were invited to apply metabolomics on a set of samples selected through a popular method of cell isolation named as sorting and we proposed to study first the effect of the process on the cell metabolome. Sorting-treated cells had an altered content of metabolites related to inflammation-like stress. The procedure also triggered alterations related to energy consumption and cell damage. Sorting-induced biochemical changes should be taken into account in the design of robust metabolic assays of cells separated by flow cytometry.
Similarly, in a study on mice hippocampus we detected the alteration of metabolites with the time elapsed postmortem until the tissue was collected, being GABA one of the most altered metabolites, which should be considered both in the design and interpretation of the experiments.
One of my favorite topics nowadays is data treatment and how it can completely modify your results. I always think about this old joke: The statistician asks to his boss: “I can prove it or disprove it, what do you want me to do?” Researcher’s responsibility is to guarantee that results are not biased, either in a voluntary or non-voluntary way.
Sometimes my students will come up with a result and they say: “I tested different normalization strategies and finally selected this one that gives the best results” and I always ask them “and does it make sense in your biological system?” They usually look at me with wide eyes asking “what do you mean?”
I can show you an example, in an experiment we were comparing two groups of cells: control and treated – and those treated were smaller in size due to the effect of the treatment. We got the results and most of the metabolites were decreased in the treated group (Figure 1). We first normalized with the number of cells (red) almost no difference and afterwards we normalized with the protein content (green), which was much higher in the control group and results turned around to positive values, in the totally opposite direction. Which is the right value? I cannot give a short answer, but it is related to the understanding of the real biological question what is making the difference is the number or the size of the cells. A similar situation happened with heterogeneous tissues. When you compare a fibrotic kidney versus a healthy one in the same weight of damaged tissue there are less cells, normalizing by weight or by proteins will give a different result. You need to decide which is the relevant factor in your study.
Figure 1: comparison of data statistics
Another important point is metabolite identification, once you select a signal with statistically significant weight you must identify the compound under that signal, but often researchers tend to select the identification that better matches with their hypothesis. Once in a PhD defense the candidate even defended that they had developed a “new methodology” according to which they selected the identification that better matched their aims! Identification requires a profound knowledge of mass spectrometry and long hours spent in front of the spectra. Shortcuts only lead to wrong or biased decisions and literature is full of examples.
Conclusions and future directions
Nowadays technologies evolve very quickly. We are observing the growth of lipidomics, a part of metabolomics devoted to lipids, which is quite demanding in analytical terms, mainly separation and identification, due to the number of lipid isomers. In parallel, a growing number of databases are appearing and new separation techniques such as ion mobility are adding a fourth dimension to our identification capabilities: collision cross section.
Again the temptation is there: using fancy technology to get quick results. However, as I said there are no shortcuts, a deep learning of adduct formation and fragmentation patterns in mass spectrometry and the concepts of ion mobility will permit the researchers to open the black box and make real and amazing discoveries.
The opinions expressed in this feature are those of the author and do not necessarily reflect the views of Bioanalysis Zone or Future Science Group.
Our expert opinion collection provides you with in-depth articles written by authors from across the field of bioanalysis. Our expert opinions are perfect for those wanting a comprehensive, written review of a topic or looking for perspective pieces from our regular contributors.
See an article that catches your eye? Read any of our articles below for free.