Machine learning to predict drug approval likelihood prior to clinical trials
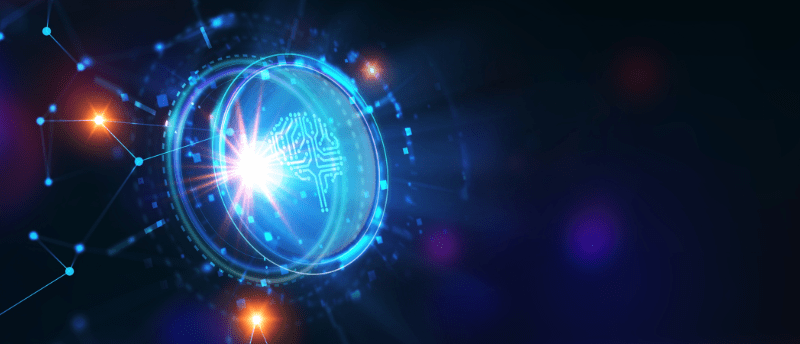
A research team headed by Professor Sanguk Kim from the department of Life Sciences, School of Convergence Science and Technology (Gyeongnam, South Korea) and Ph.D. candidate Minhyuk Park from the department of Life Sciences, Pohang University of Science and Technology (Gyeongsangbuk-do, South Korea), used machine learning to predict potential drug outcomes before the initiation of drug clinical trials, allowing them to estimate and assess any adverse effects that could occur. Their results were published in the journal EBioMedicine.
The drug development process is commonly confronted with various difficulties in human clinical trials, even after a successful demonstration of safety and efficacy in cell and animal models. A small, solitary obstacle can lead to major financial losses. Therefore, it is crucial to gain a comprehensive understanding of why certain drugs, despite successfully navigating the preclinical phases, can encounter difficulties in the clinical trial stage. This has placed a great emphasis on the need to predict the success rate of a drug’s approval.
Before human clinical trials, drugs are predominantly evaluated using cell lines and animal models. Nevertheless, the differences seen in the functioning and expression of drug target genes between cells and humans can result in discrepancies in observed drug effectiveness or toxicity. Overlooking these discrepancies can result in unexpected and severe side effects in patients, deviating from the laboratory findings.
To assess this disparity and predict drug approval outcomes, the team analyzed the knockout of CRISPR-Cas9 and evaluated the effects of loss-of-function mutations in genes for humans. This was conducted with a total of 2474 drugs, 1404 being approved and 1070 being unapproved. To confirm the risk associated with drug targets exhibiting this cellular/human discrepancy, the research team assessed the targets of drugs that failed or were withdrawn from the market due to safety concerns.
The team developed a machine-learning method to predict drug approvals during clinical trials. Unlike traditional approaches that mainly rely on a drug’s chemical properties while disregarding genetic distinctions between cells and humans, the team fused both chemical and genetic techniques to enhance the precision of their predictions regarding drug safety and success. Kim explained:
‘’The challenges of drug development rose from the absence of reliable methods for predicting clinical trial outcomes in humans. I hope our research enables us to effectively predict drug approval possibilities, substantially reducing the time and expenses associated with drug development.”