Implementing AI-driven digital transformation in bioanalysis
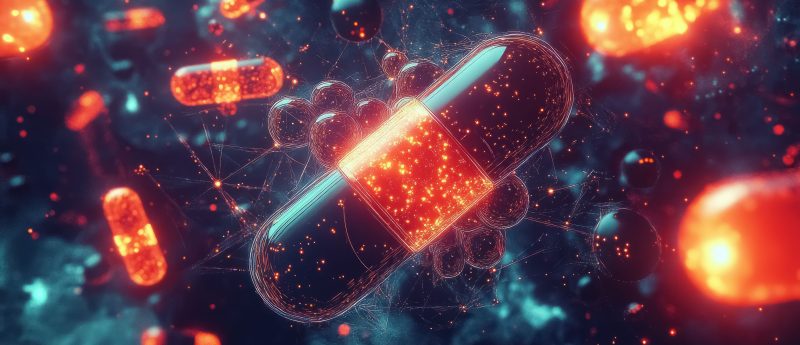
In this expert opinion, Seema Rajaram discusses how AI is revolutionizing bioanalysis by enhancing sample testing, predictive analytics, smart instrumentation and data integration, while also highlighting the strategic, technical and regulatory steps needed for successful adoption. Seema also addresses challenges like data consistency, cultural change and ethical considerations in deploying AI-powered systems within bioanalysis.
Bioanalysis is going through a major digital revolution as the life sciences industry develops, primarily because of artificial intelligence (AI). This change is improving drug development and biomarker discovery’s accuracy, speed and scalability, in addition to updating laboratory procedures. Adopting new technologies is only one aspect of implementing AI in bioanalysis; another is a strategic rethinking of infrastructure, talent and processes.
The role of AI in bioanalysis
Bioanalysis is all about uncovering and measuring the presence of drugs, metabolites and biomarkers in biological systems – a critical step in understanding health and disease. Historically, this process was slow and heavily reliant on manual methods, often requiring painstaking attention to detail. Today, AI is rewriting the script by introducing speed, accuracy and automation. It’s not just simplifying workflows, it’s transforming bioanalysis into a seamless, data-driven powerhouse.
Key applications of AI in bioanalysis
1. Automated sample testing
AI-powered robotics are transforming how biological samples are processed and tested, taking tedious manual tasks off the table and drastically cutting down on errors. Imagine the impact companies like Dash Bio (MA, USA) are having by leading the charge with systems that shave analysis timelines from months to just weeks. This incredible efficiency allows pharmaceutical teams to make quicker, well-informed decisions during clinical trials, speeding up the path to life-saving treatments.
2. Predictive analytics & machine learning
Machine learning algorithms work behind the scenes, analyzing both historical patterns and real-time data to spot trends, detect anomalies and anticipate equipment failures. By taking a proactive approach to quality control and maintenance, these systems keep processes running smoothly. In bioanalysis, this means reliable assay performance and strict adherence to regulatory standards, ultimately ensuring trust and accuracy in every result.
3. Smart bioanalytical devices
AI-powered analytical instruments are redefining how assays are conducted. By adjusting parameters instantly based on incoming data, they ensure more accurate and reliable results. These advanced systems also offer remote diagnostics and decentralized testing capabilities, making them perfect for point-of-care settings where speed and precision are critical.
4. Data integration and interpretation
AI has the remarkable ability to bring together data from various systems like ELNs, LIMS and mass spectrometry outputs, and process it at lightning speed compared to traditional methods. This capability is a game-changer, especially in complex areas like multi-omics and personalized medicine, where quick and accurate insights can lead to more targeted and effective treatments.
Strategic steps to implement AI in bioanalysis
To successfully integrate AI into bioanalysis, labs should first evaluate their existing technology and digital readiness. Ensuring structured, high-quality and FAIR-compliant data is essential. Selecting scalable AI tools that align with lab objectives, training staff to embrace these innovations, and fostering a culture of adoption are key steps. Additionally, implementing Explainable AI (XAI) frameworks ensures transparency and regulatory compliance, paving the way for a seamless and effective transition to AI-powered workflows.
Challenges to address
Inconsistent data formats can limit AI’s effectiveness, while evolving regulatory guidelines leave uncertainty around bioanalytical validation. Transitioning from manual processes to AI-augmented workflows requires time and a cultural shift, and ethical concerns demand AI models that are transparent and free from bias to ensure trust and fairness.
Final thoughts
Bringing AI into bioanalysis is a game-changer, making scientific processes faster, smarter and more accurate. While adopting this technology involves both operational adjustments and cultural shifts, the payoff of quicker timelines, deeper insights and better results is hard to ignore. Rather than replacing scientists, AI gives them the tools to innovate and make an even greater impact.
Disclaimer: the opinions expressed are solely that of the authors and do not express the views or opinions of their employers, Bioanalysis Zone or Taylor & Francis Group.
About the author:
Seema Rajaram is a global test and validation leader specializing in digital transformation and artificial intelligence within the pharmaceutical industry. With over 18 years of experience in IT, she oversees complex global initiatives, leveraging AI and data science to enhance drug discovery and regulatory compliance processes. Her work focuses on developing and implementing advanced testing frameworks for AI-driven systems, ensuring data integrity, reliability and operational efficiency. Rajaram also plays a crucial role in risk management and security testing, protecting sensitive patient data and intellectual property in the digital pharmaceutical landscape. You can connect with Rajaram on LinkedIn.
Our expert opinion collection provides you with in-depth articles written by authors from across the field of bioanalysis. Our expert opinions are perfect for those wanting a comprehensive, written review of a topic or looking for perspective pieces from our regular contributors.
See an article that catches your eye? Read any of our articles below for free.