Flow cytometry: data lifecycle and automated gating
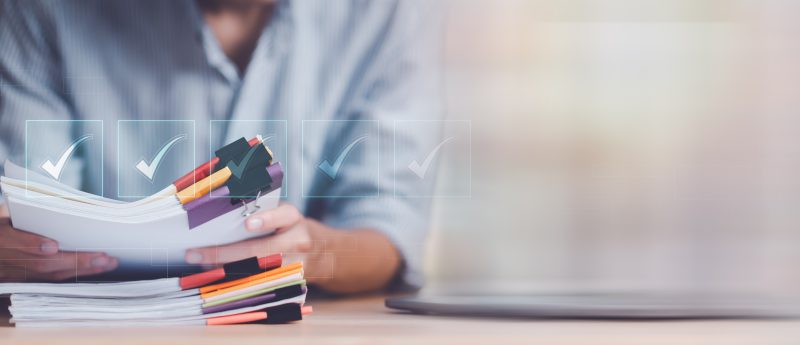
Satnam Surae PhD, Chief Product Officer, Aigenpulse (Oxford, UK)
Satnam has been active in the life sciences for more than 10 years. While originally focussing on Biochemistry, he discovered early on his passion for applying information technologies to biological challenges. His unique ability to transition between both worlds enables our teams to effectively develop the product and at the same time provides him with the ability to shape our customer engagement.
email: [email protected]
-
Could you briefly introduce yourself and your background?
I’m Satnam Surae and I’m Chief Product Officer for Aigenpulse. We enable life sciences companies to benefit from advanced data expertise and apply this knowledge across the research environment. This allows pharmaceutical and biotechnology organizations to fully focus on the output of their drug discovery and development pipeline, directing their resources appropriately and cost effectively to bring better drugs to more patients in less time.
I have a BSc in Biochemistry and a MRes in Computational Biology from the University of York (UK). I went on to University College Dublin (Ireland) to work on my PhD, which focused on engineering bone morphogenetic proteins as potential therapeutics for diabetic nephropathy. Prior to joining Aigenpulse in 2016, I was working in industrial biotechnology, where I was developing metabolic models to engineer microorganisms with the aim of producing chemical intermediates. I have been active in life sciences for more than 13 years and am passionate about creating game-changing solutions at the intersection of science, business and technology leveraging data science and machine learning to accelerate our understanding and engineering of biology.
-
What are the basic principles of flow cytometry and why do you believe it is such an important technique, being critical in pharma research?
Flow cytometry is one of many analytical techniques used in pharma R&D to develop pharmaceutical compounds. It has been used commercially in research and clinical labs for about 40 years now; primarily to investigate disease aetiology and alterations in immune responses, as well as for quantitative pharmacokinetic studies.
Flow cytometry collects complex information as streams of cells in suspension pass through a focused laser. As particles are exposed to the laser, they scatter light and any fluorochromes used to label the cell fluoresce. Both signals are detected, reflecting the physical and biological properties of the cell. By using multiple fluorochromes with different emission spectra, many data points can be captured simultaneously for every event detected. Technological developments over recent years have resulted in the availability of high-throughput flow cytometric approaches, extending applications in cell-based assays such as cell proliferation, differentiation, cell death, adhesion, ligand binding, transport and cellular signaling [1].
The high-speed quantitative analysis of cells and particles enables rapid drug molecule screening, and it is that which makes flow cytometry such an appealing, exciting technology for drug discovery research. Also, its multiparameter capabilities generate a range of different types of information – from elucidation of mechanisms-of-action (for drugs and disease progression), to functional assays, which means flow cytometry has an important role in the prioritization, verification and clinical validation of new biomarkers [2].
However, this ability to measure so many parameters means that huge quantities of data are being generated all the time, often causing a bottleneck in research. Significant computational power is required if researchers are to capitalize on the benefits of flow cytometry.
-
Could you give a brief overview of the flow cytometry data lifecycle?
The steps involved in the flow cytometry data lifecycle can be grouped into the following stages:
- Data acquisition: Importing flow cytometry data from multiple sources ready for analysis
- Processing (QC and gating): Interrogating data to remove low-quality events and tune parameters. Users can complete either manual or automated gating (see explanation below)
- Sub-population selection: Analyzing populations/hierarchies to interrogate population to parent ratio
- Results integration: Gathering the data from experiments to be able to extract insights
- Data analytics: Processing the data generated and using visualization tools
- Insight generation: Using the data to generate useful insights for research
-
Could you explain the differences between manual and automated gating? From your experience, what is your preferred approach?
Flow cytometry data analysis is built upon the principle of gating, which is necessary for the visualization of correlations in multiparameter data. Populations of interest are sequentially identified and refined using a panel of fluorochromes conjugated to antibodies that target a specific protein (marker). The fluorescence detected in the unique emission spectrum of the fluorochrome is therefore proportional to the amount of the marker present on the cell.
Manual gating is the traditional approach used by many labs but is time-consuming and can only be completed accurately by users with sufficient experience of the technique and knowledge of the biological processes at play. A key weakness in manual processes is the subjectivity in interpreting results, with user bias playing a part in the conclusions drawn from data. There has also been some reluctance to move to computational approaches due to the biological interpretability of results – gated populations are not always representative of the biology and can be difficult to match with manually gated data.
Automated gating is based on the mathematical modeling of the fluorescence intensity distribution of particle populations [3]. As well as drastically reducing analysis time, automated gating addresses the challenge of subjectivity in manual methods, and could even lead to the discovery of novel, biologically relevant populations that had not previously been considered.
However, despite the growing adoption of automated methods, manual gating has been used for many years and is the standard approach by many labs due to traditionally small-scale experiments with low numbers of markers. There is also a challenge with random variation in automated clustering algorithms, which can sometimes lead to inconsistent results if not handled appropriately. Comparing results from automated gating with each other, as well as with traditional manual gating results, has therefore been an ongoing challenge, as every new algorithm developed is assessed using distinct datasets and evaluation methods [4].
So, although a range of software platforms exist that enable automated gating, there is a lack of tools available that enable data sharing. There is therefore a need for a solution that allows gated and analyzed data to be exported from one platform and imported into another, to reproduce analyzes from raw files and facilitate demonstrable reproducibility. The CytoML Suite from Aigenpulse addresses this need by automating end-to-end processes for large numbers of raw files, by leveraging usable machine learning to empower cytometry processing and analytics.
-
Flow cytometry is one method that generates an enormous amount of data – what are the general challenges associated with big data and what are some of the solutions to overcoming this bottleneck?
In life sciences, the rapid digitalization of R&D creates vast amounts of big data and many organizations are only scratching the surface of how to organize, mine and derive value from it. The core strengths of most researchers lie in their scientific expertise, not in structuring, organizing and managing data. Yet the ongoing technological development and widespread adoption of advanced research equipment leads to a rapidly increasing stream of digital research results.
In a typical biotech/pharma company, data sources are broad in size and scope, including flow cytometry, genomics, transcriptomics, epigenomics, proteomics, metabolomics, molecular imaging, enzyme-linked immunosorbent assays (ELISA), population studies and clinical or medical records. To derive meaning and organizational value from such large, diverse, complex and dispersed datasets requires a total rethink of the way R&D is managed.
The use of one common platform that aggregates, structures and digitalizes workflows across an organization can unlock the potential of that data, providing easy access and opportunities for greater and more productive cross-department collaborations. For example, with the Aigenpulse Platform, we are lowering the entry barrier for customers to digital transformation and enabling users to leverage true machine learning on their organization’s data, augmented with public and external assets. The result is a data repository that becomes an organization’s single source of truth, where all critical information is aggregated, stored and easily accessed.
-
How do you hope to see flow cytometry being used in the future?
Pharmaceutical R&D generate so much data, which holds enormous opportunity for the development of life-changing therapies. It will be leveraged with appropriate data analytics and advanced machine learning to unlock insights and facilitate decision making. For example, more value can be derived from integrating flow cytometry data with both in-house and public single-cell, proteomics and transcriptomics data and performing deep exploration driven by machine learning algorithms. Researchers can rapidly explore large data assets to drive development decisions, and use the time saved on laborious data processing for higher value-added tasks. Importantly, platforms such as CytoML enable collaboration between both computational and non-computational researchers in cytometry and will motivate reproducible analysis by helping users and reviewers validate computational and manual analyses and analysis pipelines for pharmaceutical research.
Visit www.aigenpulse.com for more information.
References:
[1] Flow cytometry: breaking bottlenecks in drug discovery and development. Drug Target Review (2016); www.drugtargetreview.com/article/14512/flow-cytometry-breaking-bottlenecks-drug-discovery-development/
[2] Millán O and Brunet M (2015). Flow cytometry as a platform for biomarker discovery and clinical validation. In: Preedy V., Patel V. (eds) General methods in biomarker research and their applications. Biomarkers in disease: methods, discoveries and applications. Springer, Dordrecht.
[3] Montante S, Brinkman RR. Flow cytometry data analysis: recent tools and algorithms. Int. J. Lab Hematol. 41(Suppl. 1), 56–62 (2019).
[4] Aghaeepour N, Finak G, Hoos H et al. Critical assessment of automated flow cytometry data analysis techniques. Nat Methods. 10(3), 228–238 (2013).
The opinions expressed in this feature are those of the author and do not necessarily reflect the views of Bioanalysis Zone or Future Science Group.
In association with