Discovery technologies for the multi-omics era of precision medicine: an interview with Dr Mo Jain
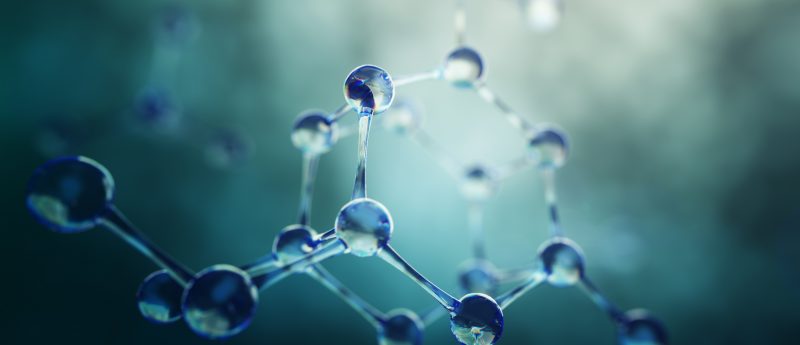
Despite significant advances in genetic sequencing over the last decade, we still understand an exceedingly small percentage of the total human system, as the majority of disease risk is actually attributable to non-genetic factors. Large-scale profiling of dynamic biomarkers can grow our understanding of human biology, health and disease beyond the genome using an integrative multi-omics approach. In this interview, Mo Jain discusses how next-generation mass spectrometry-based methods are allowing the measure of a greater breadth of human chemistry at greater speed, enabling rapid nontargeted small molecule biomarker discovery.
Dr Jain is a physician-scientist with 20+ years of expertise in physiology, biomedicine, computational biology and mass spectrometry-based metabolomics. Prior to founding Sapient (CA, USA), he formed and was Director of Jain Laboratory at the University of California San Diego (UCSD; CA, USA), where he led a multi-disciplinary research team to develop next-generation mass spectrometry-based systems to rapidly probe the non-genetic landscape of disease. He founded Sapient in 2021 as a spin-out of the lab to expand upon the mission of accelerating human discovery with large-scale discovery metabolomics.
-
Could you introduce yourself and how you came to establish Sapient in 2021?
Of course, I would be happy to do so, and thank you for the opportunity to share our story today! My name is Mo Jain and I have the pleasure of leading the Sapient team as Founder and CEO. By way of background, I trained as a physician-scientist with my MD work in adult cardiology and my PhD in molecular physiology. I did all my training in the Boston programs – at Boston University (MA, USA), Harvard Medical School (MA, USA) and Broad Institute (MA, USA) – and subsequently came out to San Diego to establish my academic program at UCSD in 2013. During that time, we began developing new technologies to innovate mass spectrometry in ways that could allow us to interrogate human biosamples with unparalleled breadth and depth. As a practicing physician, I saw the need for this firsthand: for most human diseases, we have very limited testing that enables us to diagnose diseases at its earliest time points or testing that allows to us understand individuals with a given disease, who may respond best to medicine one versus medicine two. This is the basis of what we term ‘biomarkers’ in medicine.
The very aim of our academic lab was to develop new technologies that allowed us to measure these biomarkers in human blood at a level never before done. For instance, when you go to the doctor every year, they draw two tubes of blood and measure 12–20 biomarkers or so in that blood sample. Those biomarkers tell us about the health of your heart, kidneys, liver, and other organs and biology. However, there are 12,000–20,000 biomarkers floating around in your blood at any given moment. Our goal was to be able to capture and measure these thousands of missing biomarkers to advance human diagnostics. As we were developing these tools in our academic lab, it became apparent that to release their scientific potential, we would have to provide access to these cutting-edge approaches and the data they produce. We realized that we couldn’t effectively support scientists developing and marketing medicine in the biopharmaceutical industry from our academic position, leading to the creation of Sapient in 2021. Today, we leverage our next-generation mass spectrometry systems, developed over the last decade, to support biopharma sponsors in accelerating their drug development programs, by enabling rapid, large-scale discovery of dynamic biomarkers of health, disease and drug response.
-
Can you describe the landscape of multi-omics integration in drug development today and how you see it evolving over the next 3–5 years?
Over the past decade, biomarker discovery has been critical to drug development. However, the vast majority of this discovery has focused on genomic markers. When you actually look at disease risk across common diseases, only 15–20% of risk of any given disease is attributable to genetics. Given the dynamic nature of disease, there is a need to extend to much more dynamic multi-omics processes to identify new targets and align individuals with their disease states. This is particularly important for precision medicine and the drug development process in general. Over the next 3–5 years, we anticipate a major evolution in this space, where the focus will shift from genomics to next-generation or beyond-genomics technologies, including metabolomics, lipidomics, proteomics and other such dynamic measures that are central to human health, disease and drug response.
-
How are your mass spectrometry-based technologies supporting this transformation? What are the key features enabling rapid small molecule biomarker profiling at scale?
Enabling the transition to the next era of precision drug development and personalized medicine requires technologies that are both robust and scalable. Robust in that the data they generate is highly accurate and precise, and scalable in that they can be applied efficiently across large populations. We’ve learned from the genomics revolution that technologies cannot be applied for discovery on small populations. We need large-scale datasets to gain the statistical power for a robust discovery process, especially when using humans as the model system for which discoveries are being initially made.
Sapient has been an integral part of this transformation through the establishment and optimization of our next-generation mass spectrometry technologies, which are able to go 100 times faster than a traditional mass spectrometer while also measuring five times the number of molecules typically captured. Much in the way parallelizing sequencing allowed for the measure of genetic variation across large populations at a much faster pace, we set out to develop mass spectrometry technology that could rapidly measure more of the biology that doesn’t specifically come from the genome. Today, our rapid liquid chromatography–mass spectrometry (rLC–MS) systems can measure 15,000 small molecule biomarkers in a biological sample, in under 1 minute per sample, giving us the capacity to handle thousands of samples per day for analysis.
It has taken much development innovation to fully leverage the power of mass spectrometry at speed, not just from our side but also from the advances in mass spectrometry technology by the industry at large in recent years. The introduction of high-resolution instruments that are extremely robust has been a gamechanger. We’ve been very excited to leverage the Bruker timsTOF Pro 2 in our platform, which uses trapped ion mobility as an orthogonal separation and enables reproducible measurement of collisional cross section (CCS). If you think of the mass spectrometer as the engine powering the car, the timsTOF has us racing at F1 speeds.
-
These technologies are generating a massive amount of data. Can you describe your statistical approaches and how you handle large amounts of biomarker data to identify key signals and associate them with other -omics data?
One of the key learnings over the past decade is that the goal is not data generation, but rather, knowledge generation. While these sound like they may be overlapping Venn diagrams, they are not. Whereas data generation has scaled exponentially over the last decade, knowledge generation continues to plod along at a very linear pace. The bridge between data and knowledge is bioinformatics, and the ability to take very complex data and apply statistical models and approaches to derive actionable insight. This is what ultimately unlocks the potential of these types of biomarker data. When done correctly, as we’ve seen over the last several years in the genomics world, it can be transformative in identifying new targets, sub-stratifying patients and bringing new drugs to market that are more efficacious.
This is why at Sapient we’ve focused not only on next-generation technologies to generate large-scale datasets, but also on the ability to handle and integrate very complex, multi-dimensional multi-omics data. We’ve built an in-house data science team with the expertise to leverage diverse biocomputational approaches, from Bayesian statistics to machine learning to AI, to integrate complex information from metabolomics, lipidomics, proteomics and genomics in a way that allows us to unlock data insight as quickly as possible.
-
How many of the molecules that are captured by your mass spec systems are known versus unknown molecules? When you find a novel molecule, how do you go about identifying it?
One of the great challenges, and at the same time great opportunities, of small molecule biomarkers is the sheer amount of information integrated in these measurements. 99.9% of genomic or proteomics information present within a mammalian cell comes from the mammalian host; with small molecule biomarkers, this is quite different. They not only come from our cells, but also from the world around us. Everything we eat, drink, smell, smoke, the microbes that inhabit our skin and our gut – it all results in the production of small molecule biomarkers that are integrated within our blood. Since these are not derived only from the human cell itself, no reference set that tells us exactly what these markers are. In fact, when we look across large populations, we find that the vast majority of small molecule biomarkers are unknown. Currently, at Sapient, we can capture more than 15,000 small molecule biomarkers per human biosample and can identify somewhere on the order of 1000 known biomarkers using pure commercial standards from our library, which we are continuing to grow.
While the remaining molecules we capture are unknown, one of the real paradoxes is that oftentimes the most important molecules are unknown. Therefore, we need a means by which to identify unknown but biologically significant molecules we discover. We can do this through a number of approaches, for example using computational models for structural prediction that allow us to then proceed immediately to organic synthesis, or using tools to isolate the molecule out from pooled samples for NMR identification and ultimately synthesis.
It is also important to remember that even in the absence of identifying the structure of an unknown molecule, because their chemical properties are defined on our mass spectrometry systems, we can follow these from one experiment to the next to validate them. We can begin the development of a CLIA-based assay around an unknown marker even in the absence of structural information. This is one of the great advances over the last several years. You can think of metabolite identification as a parallel track to advancing a particular marker as part of a drug development program. It’s no longer a serial limitation.
-
How are you extending the use of these systems? For example, do you have plans for proteomics?
Although our focus has largely been centered on small molecule biomarker discovery to date, there are many, many different types of biomarkers and molecules that can be assayed using our technologies. We are in the process of developing pipelines for discovery proteomics, which is heavily mass spectrometry-based, as well as precision and targeted proteomics approaches that leverage both mass spectrometry in tandem with other technologies like binding agents to really interrogate proteins of interest. Bruker has played an integral role in helping us leverage the timsTOF systems we already have in place for these workflows, and we’re really quite excited about our ability to integrate metabolite, lipid and protein measures together with genomic information. We feel that this will reveal much more about the complexities underlying human biology and greatly advance forward actionable insights for our sponsors and clients to leverage in their programs.
-
Looking towards the future, what are you most excited about in the field of precision medicine?
Precision medicine continues to be an area that is evolving very, very quickly. I believe there are several lessons we learned as a scientific community, and frankly as a society, through COVID-19, including just how important it is to be able to develop and deploy drugs, whether they be vaccines or therapeutics, at a much faster rate and on a global scale. The only way in which this works at scale, given the high cost of drug development, is through precision-guided approaches. This is what truly excites me for the future; thinking about how we can begin to leverage the types of data we’ve generated, not only for target identification, which is where most of this work has really centered over the last decade, but also extending it to preclinical toxicology, to early guidance for sub-stratification of patients and to early diagnosis.
Ultimately, using these insights to develop and deploy drugs in a much, much more precise manner will allow us to consider an individual patient, understand their specific disease process and then best apply a drug for which their specific disease will have a response. When we’ve seen this work, for instance, in the oncology space with targeted therapeutics, it can be transformative. Now it is high time that this be extended from very rare tumors to the general population and the most common diseases.
In association with