Data governance in life sciences: building solid data foundations to address key challenges
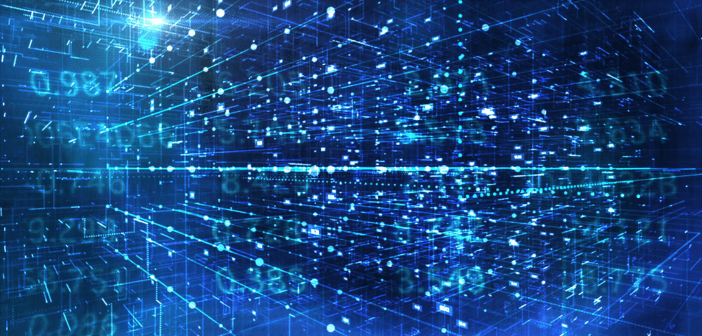
Opportunities for life sciences organizations to collect and analyze health data are growing exponentially due to the rise in technologies such as software as a medical device (SaMD) and the adoption of wearable tech.
For the past 5 years artificial intelligence (AI), and specifically AI-enabled technologies, have been transforming the different parts of the biopharma value chain, from drug discovery to post-market surveillance, creating new business opportunities [1]. The latest disruption offered by generative AI – which can support data-driven insights and discoveries faster than ever before – adds another ripple to the wave of disruption due to its ability to analyze large datasets, uncover hidden patterns and generate valuable insights that would otherwise remain undiscovered. It also has the potential to equip employees with tools that can significantly increase their productivity. These new technologies are fundamentally fed by existing data and help to generate new data, so it is even more crucial to manage and govern that data effectively.
The Summer 2023 Fortune/Deloitte CEO survey reported that ‘while still early in its adoption, 79% believe generative AI will increase efficiencies and half (52%) believe it will increase growth opportunities’ [2]. Leaders in the data-driven biopharma industry recognize that unlocking use cases across the value chain is important but acknowledge the need for compliance with new regulations designed to protect data and patient privacy. How can effective governance of data play a key part in supporting organizations to capitalize on their use cases? And how can organizations approach becoming ‘role models’ for data governance (DG), through a people, process and technology lens?
What is data governance?
DG is a set of quality control processes that help in managing, using, improving, maintaining, monitoring and protecting data across the organization. DG helps organizations comply with both general regulations (e.g., the General Data Protection Regulation (GDPR), Health Insurance Portability and Accountability Act (HIPAA)) and regulations from industry agencies such as the Food and Drug Administration (FDA), the European Medicines Agency (EMA) and the Medicines and Healthcare Products Regulatory Agency (MHRA), concerning data collection, storage and usage in accordance with country laws.
DG is increasingly being seen as a pre-requisite to becoming a truly data-driven organization, and a catalyst to extracting commercial value and innovation opportunities from data. Specifically, in the life sciences industry, DG is foundational for organizations to use their vast collection of clinical data, including genomics data, real-world evidence, operational data and commercial data to make smarter decisions to support key business processes. This includes indication planning, patient recruitment, trial supply planning, regulatory submissions and commercial positioning of their drug assets.
DG should be viewed as an enabler, encouraging a range of benefits such as greater efficiency, more innovation and insights and higher cost savings through more effective use of data. It can be a driver for:
Data-driven decision making – unlocking the full potential of an organization’s data and analytics capabilities and empowering them to make more informed decisions;
Improved reputation and increased trust in data – developing policies and procedures to ensure that data remains compliant with regulatory standards, securely stored and used only for intended purposes;
Healthy data culture and data literacy – empowering the workforce through training and upskilling to effectively understand, analyze and communicate with data;
Optimized data sharing and accessibility – establishing data standards to optimize data sharing and reduce duplicative work;
Reducing operating costs and improving operational excellence – creating DG processes for up-to-date data ensures accuracy, reliability, compliance and security.
Industry outlook: role of data in use cases across the life sciences value chain
The life sciences industry strives to increase the speed and efficiency of how it navigates regulatory submission and brings its products to market to address patient needs whilst also responding to the industrialization and adoption of digital technologies. Industry research by Deloitte Research (London, UK) has identified a list of priority use cases that teams across the life sciences value chain can pursue. Data plays a fundamental role in enabling these use cases (see Figure 1).
Figure 1: Data-related use cases across the life sciences value chain [3].
Extracting value from data such as historic randomized clinical trial datasets, operational datasets, real-world evidence datasets and manufacturing and supply chain data requires investment in cataloging, management of master data, policies and procedures to access the data and monitor its quality. These are capabilities that organizations that we have identified as ‘role models’ [4] of data governance ensure their people have. Figure 2 describes the crucial role of DG as a foundational set of capabilities that are needed in enabling data use cases across the life sciences value chain.
Figure 2: The role of data governance across the life sciences value chain [5].
What happens when data governance is neglected?
Poor DG can have a significant negative impact on a business’s bottom line, productivity and reputation. Ensuring that an organization’s data is of sufficient quality and aligns with findable, accessible, interoperable and reusable principles [6] is challenging. Organizations need to develop strategies to overcome these challenges. It is therefore important to implement DG practices that enable the most effective use of data and ensure that it is protected, accurate, reliable and secure.
The importance of data integrity
The integrity of data generated by highly regulated life sciences companies is critical because properly recorded information is the basis for manufacturers to assure product quality, safety and efficacy prior to product approvals and subsequently placing them onto the markets for human use. Data integrity is also important for quality control procedures during manufacturing to ensure patient safety. As global regulatory focus on data integrity increases, companies that fail to comply may face penalties ranging from public warning letters and product removal from the marketplace to criminal charges. In recent years, there has been a significant increase in the number and types of issues related to data practices, including unauthorized data access, lack of enabled audit trails and accidental and intentional falsification of records. Regulatory bodies now have high expectations when it comes to data quality and integrity. This is in part testament to the life sciences industry’s growth, globalization and adoption of advanced technology, such as highly automated systems and storage of data in the cloud. The need to be compliant is expected to drive organizations to make significant changes to their current data-related processes and systems that require corporate-wide efforts and cross-functional collaborations. The implementation of good data practices requires consideration, not just of controls, processes, IT and clear roles and responsibilities, but of a wider shift towards education and a culture that understands and values data integrity.
Based on our research, observations and client engagements, we see that our ‘role model’ organizations focus on multiple characteristics and components of people, processes and technology to build foundational capabilities and contribute to effective data governance of life sciences data.
People & organization
- Establish data governance roles and responsibilities (such as data stewards and ethics specialists) embedded within the life sciences value chain and supported through a network of cross-functional DG forums/councils.
- Gain commitment/buy-in from all business areas – consider establishing a CDO (Chief Data Officer) group to define and formalize cross-functional roles for data security, privacy, quality, ownership and management of the data. Data is not the sole responsibility of a single group.
- Set up objectives related to data quality, integrity, privacy and compliance with staff objectives.
- Invest in data literacy and upskilling/training of employees to ultimately help enable data-driven decision-making and improve data transfer between business functions.
Process and policy
- Define a data governance strategy and plan that is anchored to support business use cases and initiatives across the value chain.
- Establish processes for data acquisition, storage, ownership and cataloging that can streamline the onboarding of external data assets such as real-world data.
- Determine retention periods and end-to-end data lifecycle processes to ensure that appropriate actions are followed at each stage of the data lifecycle. This is particularly relevant for the use of patient data according to country privacy laws and regulations.
- Make strategic choices to prioritize specific areas across the organization based on business needs, while ensuring that lower-priority initiatives are not neglected in the long run.
Technology and tools
- Invest in tools to support data cataloging, data lineage, data quality and master data management to help build trust and transparency of data usage while adhering to GxP standards in handling patient-level data.
- Consider automating DG processes such as policy-based data access provisioning which minimizes the risk of data loss, misuse and security breaches.
- Use AI/ML to automate data quality assessments and support data classification based on patterns, content and context to help organize data and improve findability.
Role model organizations show best practices when they embed DG into their ways of working. This is typically guided by a federated DG group which can advocate the importance and value of DG in each part of the business, enabling them to govern and manage their own data. It is important to highlight the business value of data governance to everyone within the organization, both top-down and bottom-up, to promote the adoption of good data practices and tools across the life sciences value chain. Strategic decisions should be made specific to each organization’s business needs, challenges and priorities, as well as industry requirements and best practices.
Disclaimer: The opinions expressed in this feature are those of the author and do not necessarily reflect the views of Bioanalysis Zone or Future Science Group.
References:
- Deloitte Insights. AI in Biopharma. (2023): https://www2.deloitte.com/uk/en/insights/industry/life-sciences/ai-in-biopharma.html
- Deloitte. Summer 2023 Fortune/Deloitte CEO Survey Insights. (2023): https://www2.deloitte.com/us/en/pages/chief-executive-officer/articles/ceo-survey.html
- Deloitte. 2023 Annual Review. (2023): Deloitte UK | Audit, Consulting, Financial Advisory and Tax services
- Deliotte. Data Governance. (2023): https://www2.deloitte.com/uk/en/pages/consulting/articles/data-governance.html
- Deloitte. Deloitte presentation on Data governance in Life Sciences. (2023): Deloitte UK | Audit, Consulting, Financial Advisory and Tax services
- Go FAIR. FAIR Principles. (2023): https://www.go-fair.org/fair-principles/