Ask the Experts: organization and transformation of bioanalytical data for PK analysis
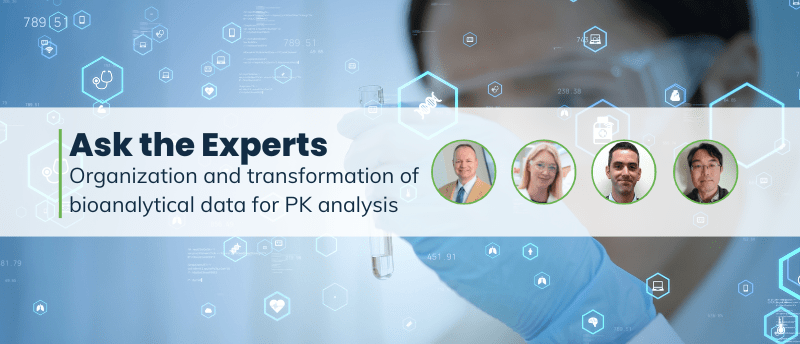
In this Ask the Experts feature, our experts discuss the ‘benchtop to desktop’ process. This refers to the organization and transformation of bioanalytical data, extracting the raw bioanalytical data from an Excel spreadsheet or laboratory information management system (LIMS) and converting it into a useful format for PK analysis.
Meet the experts
Sheridan Jaeger
Associate Pharmacokineticist
Alturas Analytics (ID, USA)
Sheridan Jaeger is an Associate Pharmacokineticist and Technical Writer at Alturas Analytics. She is a graduate of Washington State University (WA, USA) and joined Alturas in 2019. Her primary focus at Alturas is PK/TK analysis and she works as the Principal Investigator for regulated and non-regulated animal studies. Sheridan loves interacting with clients and being part of a team that helps fight diseases.
Peter Bonate
Executive Director of the New Technologies Group
Astellas (IL, USA)
Peter Bonate is the Executive Director of the New Technologies Group within Early Development at Astellas. He has more than 25 years of industry experience in pharmacokinetics. He is a Fellow of the American College of Clinical Pharmacology (VA, USA), a Fellow of the American Association of Pharmaceutical Scientists (AAPS) and a Fellow of the International Society of Pharmacometrics (ISoP). He is currently Editor-in-Chief of the Journal of Pharmacokinetics and Pharmacodynamics and Associate Editor of Pharmaceutical Statistics. He is also the author of the book ‘Pharmacokinetic–Pharmacodynamic Modeling and Simulation, 2nd edition’, which has had over 50,000 chapter downloads since its publication.
Kim Deadman
Pharmacokinetics Team Lead
Resolian (Fordham, UK)
Kim joined Resolian (formerly LGC) in 2016 and is currently the Pharmacokinetics Team Lead, responsible for managing the reporting and delivery (including SEND and SDTM) of pharmacokinetic analysis for pre-clinical and clinical studies. After graduating from the University of York (UK) in 2003 with a BSc in Biochemistry, Kim began his career at GlaxoSmithKline (Ware, UK) where he developed skills in bioanalysis and toxicokinetics (TK) over a period of 9 years. Subsequently, he joined the Monash Institute of Pharmaceutical Sciences (Parkville, Australia) where he specialized in method development for bioanalytical assays and provision of mass spectrometry training in support of the Inhaled Oxytocin Project and HMSTrust Analytical Laboratory (Melbourne, Australia).
Makoto Niwa
Principal Scientist, Drug Metabolism & Pharmacokinetics Research Division
Nippon Shinyaku (Kyoto, Japan)
Makoto is a Principal Scientist in the Drug Metabolism and Pharmacokinetics Research Division of Nippon Shinyaku. Makoto also worked as the Pharmacokinetics Team Lead at Nippon Kayaku (Tokyo, Japan) and has 30 years of experience in pharmacokinetics and bioanalysis, supporting projects from drug discovery to new drug approval. He is currently responsible for the management of discovery bioanalysis. During his career as Staff Scientist and Study Director, Makoto was responsible for analytical method development using mass spectrometry, performing analytical runs, data processing and reporting. He majored in agricultural science with an emphasis on analytical chemistry at the University of Tokyo (Japan), where he received his M.Sc., and later completed his Ph.D. at Ritsumeikan University (Kyoto, Japan), with a focus on management of technology.
Questions
Sheridan: For nonclinical PK/TK studies, the first step is to gather all the information needed for analysis: concentration data, nominal collection time and animal identification from the bioanalytical dataset; dosing and group information from the protocol; actual sample collection dates/times from the in-life facility; and any other information that could affect analysis such as early terminations or dose excursions. Then the data is transformed, merging all of the required variables into one dataset, and normalizing time units from minutes to hours. Additionally, samples below the limit of quantitation are transformed according to the LLOQ imputation rules stated in the protocol or per our standard practices.
Peter: The New Technologies and Pharmacometrics Groups at Astellas use clinical and preclinical analytical data, both PK and biomarkers, in many ways. We develop mechanistic models of drug effect, physiological-based pharmacokinetic–pharmacodynamic (PD) models, population PK–PD models (PopPKPD), or analyze the data using noncompartmental methods (NCA). Data organization and transformation are at the heart of what we do as modelers. An often-overlooked aspect of modeling is data wrangling, the process of transforming the data from multiple, often differently formatted datasets into a single, unified dataset suitable for data analysis. Getting the data into the right format is essential before any of the real work of modeling can begin. Long before any modeling is done, the modelers interact with the programmers to ensure that when the data becomes available, it is in a format that can be used without any further delays. To this end, we have data standards in place for many of our activities, like NCA or PopPKPD. Programmers use these standards to develop the code that we will ultimately analyze.
Kim: We receive concentration data from various sources, both within our organization and from external organizations, which come in various formats. We may also receive separate datasets containing dose administration information, randomization schedules and laboratory test results. These datasets usually require transformation to allow them to be merged prior to analysis. We make further transformations to apply below the limit of quantitation (BLQ ) rules, remove controls, assign or change units and deal with any study deviations that may impact the analysis. We may also perform other preliminary calculations, for example, calculation of actual sampling times, urine volumes, epithelial lining fluid volumes using urea ratios and dose calculations for inhalation studies.
Makoto: In exploratory pharmacokinetic activities, information on how the sample was processed is minimal, as the process is standardized. Data to be collected include sample name, calibration standard, sample responses (i.e. peak area) and quantitated concentrations. In more sophisticated studies in the drug development phase, sample processing information including sample dilution and dilution factor in quantitation should be noted. Such information is provided from a laboratory notebook. In PK studies, quantitated concentrations are submitted to calculate PK parameters. For this, PK sampling time and sample concentration are merged. Transformation of time period data is necessary and the concrete method depends on the standard procedure of the test site. I largely use a combination of Excel® and WinNonlin™ in nonclinical PK settings, and in such a case I use the TIME MS Excel worksheet definition for the ability to use Excel functions. It is very important to use Excel in a Nonlin-ready format, i.e., by putting one-dimensional data in a column with sorting information in another column, from the beginning of the process, rather than using Excel in a two-dimensional table format. Well-designed Excel datasheets can be saved as CSV (comma-separated values) files for other processing techniques.
Sheridan: The bioanalytical sample analysis is extensively reviewed by our Quality Control (QC) department, and in the case of regulated studies, will also be audited by our Quality Assurance Unit. The bioanalytical data is procured from our Watson laboratory information management system (LIMS) and imported into WinNonlin. We have a peer review and secondary analysis process to ensure the accuracy of the TK/PK analysis. Additionally, our QC department reviews the concentration data used for non-compartmental analysis (NCA) against the bioanalytical data in Watson LIMS to ensure the accuracy and integrity of the data. All TK/PK phase reports go through a quality control review and are audited for regulated studies.
Peter: Once the data are received from the programmers, the dataset must be reviewed for errors. Even with the best planning, the dataset may contain errors, particularly when you start to analyze data within a patient. Oral concentration–time data may show unexpected increases in drug concentrations during the terminal elimination phase. Within a time point, concentrations may be unusually large or missing when they shouldn’t be. The review process for a modeler generally starts with a graphical examination of the data. Line plots of individual data are plotted over time and then as spaghetti plots with all subjects. Datasets are reviewed for missing values specifically defined as ‘NA’ or such, but also in terms of missing rows looking at the number of observed samples per time point to ensure the expected number of observations. Once it is decided that the dataset contains errors, these are sent to the programmer to ensure the problem was not on their end and to fix the error. If the observations are truly too large or too small, the modeler will reach out to the bioanalytical scientist to verify the result. Once the result is verified, these unusual values may need to be accounted for during any type of modeling process.
Kim: All our programming steps undergo QC checks by an independent pharmacokineticist, including the import of data files into Phoenix WinNonlin, manual checks of calculations and fit of regression lines. Imported data is checked against the study protocol, for example, we check that nominal times and doses are in agreement with the protocol and study design. Both the pharmacokineticist and QC checker will also scrutinize the PK profiles for any pharmacokinetically implausible concentrations, outliers, incomplete profiles, missing data or signs of mis-dosing and other deviations. Any such issues will need to be addressed as part of the analysis. For regulated studies, handling of data, record keeping and transformations and programming are audited by our QA department.
Makoto: The data is reviewed by another scientist to detect errors. Concretely, up to 10% of extracts of the data are checked for errors in the data transfer and merge process. Transfer error checks can be done by a systematic approach. At least one data from one block of data transfer or merge block should be checked. Data reliability is confirmed based on the white-box approach. For regulated studies, the whole study process is checked by an independent QA department from the viewpoint of quality assurance, where emphasis is put on the validity of quality systems rather than quality control, i.e., the quality of work products.
Sheridan: It depends on the complexity of the study and the data. The more sort variables required, and the more sources these data are acquired from, increases the time for data transformations. Additional time is required when requesting further information from external sources. Data transformation is probably 5% of the overall analysis time for simple datasets. However, if the dataset is more complex, requiring merging data and multiple variable transformations, then the transformations may take 10–40% of the time compared to the overall analysis.
Peter: Data wrangling can take hours, days, or weeks, depending on whether data standards are in place beforehand, how difficult the original data format is upon which to build the modeling dataset, and whether the data contains many errors, which may take multiple rounds of going back and forth to the programmer before a final dataset is received. Lack of data standards will significantly prolong the time it takes to get the data into a usable format. Some data, particularly biomarker data from specialty vendors, may come in data formats like Excel that are difficult to extract and require manual coding that may be slow. How data wrangling impacts the overall analysis time depends on many factors. For some analyses, like PopPKPD, which take a long time to complete, data wrangling may be as high as 20% of the analysis time. But for NCA, which may take only a few hours to complete, data wrangling can significantly prolong timelines and may be many-fold longer than the actual analysis itself.
Kim: This is very much study-dependent. For routine pre-clinical analysis, the initial data transformations can be quick. For clinical studies where data may be imported from multiple sources, transformations take longer, and it is not uncommon to uncover errors or discrepancies when calculating actual sampling times i.e. time from dose administration to sample collection. We need to scrutinize the data as early as possible. This ensures that there is sufficient time to resolve any errors, which may involve communication across different organizations.
Makoto: In standardized analysis typically performed in exploratory PK, pure data processing makes up 5 to 10% of total time, which includes sample processing, the analytical run and data processing. In complex studies, data transformation can be a more significant part of the study.
Sheridan: We use Phoenix WinNonlin with the PK Submit plug-in for creating SEND/SDTM domains. WinNonlin allows us to track all the data transformations in a logical progression, and to set up workflow templates, which saves time for repetitive steps.
Peter: Most programmers use SAS for dataset creation. Most modelers, at least in the pharmacometrics field, use R. Some modelers still use SAS, but this is mostly for dataset manipulation. Datasets created by programmers may either be in SAS format or .CSV files.
Kim: We use Phoenix WinNonlin and its associated programming objects to perform transformations and generate SEND or SDTM domains.
Makoto: As PK analysis is usually done by WinNonlin, and because of the rich pre-processing ability of WinNonlin including BLQ rules, WinNonlin plays a central role in these transformations. In preclinical PK settings, Excel can be used to compile the data. In exploratory PK with low-budget settings, extensive use of Excel including PK VBA (visual basic for applications) macro is possible, but it is not very efficient.
Sheridan: Taking a moment to make a plan in order to achieve what the end product needs to be can help streamline analysis. Having a ‘pre-flight’ checklist to organize analysis is valuable for increasing efficiency in analysis, as it reduces the need to re-run analysis for missing data. Such a checklist may include a list of applicable datasets that will be required for creating the PK data, graphing the concentration vs. time data to ensure data alignment, and identifying outliers for exclusion. Additionally, building and using workflow templates is a big advantage in saving time when the same set of data transformation steps will be performed. These templates can be used for multiple datasets, reducing the time it takes to transform data.
Peter: Dataset standards should be put in place for routine dataset creation that is repeatedly used. These standards should harmonize the order of columns, the name of columns, the format of data within columns and how to handle missing data, as well as, data above the upper and lower limits of quantification. For one-off datasets, a data format document should be developed, which has many of the same elements as the data standard document but may also contain unique variables for a particular analysis. The programmer should generate a dummy dataset to verify that the analyst has everything they need for their analysis and that the provided format does indeed match what the analyst needs.
Kim: We streamline the formatting of data and subsequent analysis by using programming templates. We can pick from a variety of templates, each suited to a particular study design, or tailored to the requirements of a customer.
Makoto: As a heavy user of Excel, paradoxically I would say eliminating the use of Excel enables more efficient PK analysis. Initially, all necessary information including sample ID and dilution factor should be put in CDS (chromatography data system). Next, analytical results should be extracted in CSV format. Then, the analytical result and PK sampling time should be compiled based on sample ID and analyzed by WinNonlin.
In association with: