Ask the Experts: the importance of data cleaning in laboratory operations
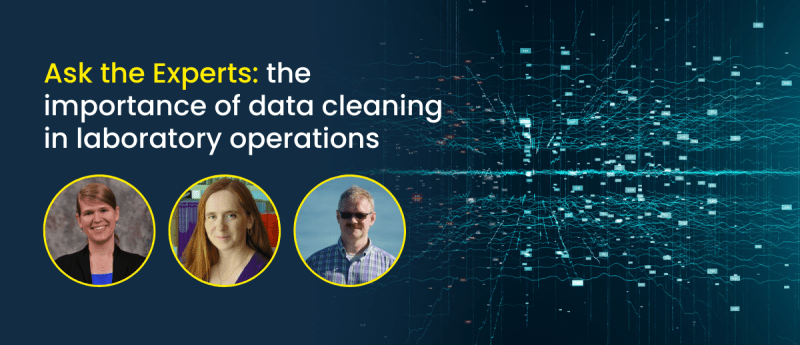
In this Ask the Experts feature, our experts discuss the data cleaning process. This refers to the process of reconciling sample metadata between the electronic data capture (EDC) system, central lab database, site records and contract research organization (CRO) database. Our experts comment on the importance of data cleaning in laboratory operations, how to streamline data cleaning, and their tips and tricks on ensuring a successful data cleaning process.
Meet the experts
Elizabeth Walker
Materials Coordinator
Alturas Analytics (ID, USA)
Elizabeth Walker is a Materials Coordinator at Alturas Analytics, Inc (ID, USA). She is a graduate of the University of Idaho (ID, USA) with a degree in Chemistry with an emphasis on pre-medicine, and she joined Alturas in 2012. Her primary focus at Alturas is sample database management. During her tenure at Alturas, she has been integral in the creation and implementation of critical processes, including cataloguing and organizing samples for active studies, post-study storage and the use of barcodes for sample tracking throughout laboratory operations. Elizabeth takes pride in being part of a team dedicated to fighting disease.
Lucia Costanzo
Research & Scholarship Librarian
University of Guelph (Guelph, Canada)
Lucia Costanzo is the Research & Scholarship Librarian at the University of Guelph (Guelph, Canada). She recently completed a secondment at the Digital Research Alliance of Canada (the Alliance) (Ottawa, Canada) as the Research Intelligence and Assessment Coordinator. As part of this role, Lucia coordinated the activities of the Research Intelligence Expert Group, which included informing and advising the Alliance RDM Team and Alliance management on emerging developments and directions, both nationally and internationally, in RDM and broader Digital Research Infrastructure ecosystems. Before the secondment, Lucia actively supported, enabled and contributed to the learning and research process on campus for over 25 years at the University of Guelph.
Serhiy Hnatyshyn
Scientific Associate Director
Bristol Myers Squibb (NJ, USA)
Dr Serhiy Hnatyshyn is currently a Scientific Associate Director at Bristol Myers Squibb (NJ, USA), leading the Automation group at the Discovery Pharmaceutics and Analytical Sciences department in the Preclinical Candidate Optimization division. Dr Hnatyshyn received a PhD in Physical Chemistry in 1996 from Ivan Franko National University of Lviv (Ukraine) and a second PhD in Environmental Sciences in 2001 from Tennessee Technological University (TN, USA). He joined the Mass Spectroscopy group in the R&D division of Bristol-Myers Squibb in 2001 and has over 20 years of experience in bioanalytical chemistry, automation, chemometrics, bioinformatics and software development. His current role is focused around automation, data analysis, software development and logistics.
Questions
Elizabeth: Data cleaning is the process of reconciling sample metadata between the electronic data capture (EDC) system, central lab database, site records and CRO database. Examples of such metadata include patient visits, collection dates/times, dosing dates/times and the location of samples between sites, among many others. The sponsor may review this information themselves, but they may also utilize the services of their central lab or hire a third party to perform the reconciliation.
When a discrepancy between the EDC and one or more other sources is identified, the data cleaning team issues queries to the parties involved to determine the true information. After verifying the true information, the data cleaning team sends corrections to the parties as needed and the various databases are reconciled. These communications usually occur in the form of correction spreadsheets that list the discrepancies, who should investigate and the updates required for each database. Data cleaning ensures that databases are reporting consistent and accurate metadata across the entire study.
Lucia: Researchers typically spend 80% of their time cleaning and organizing data, leaving only 20% for analysis. Data cleaning is important for ensuring accuracy and quality, as it involves identifying and correcting inaccurate, duplicated, incomplete, or improperly formatted data. No matter how good your methods are, the analysis relies on the quality of the data. That is, the results and conclusions of your research will be as reliable as the data you use.
Serhiy: Data cleaning involves integrating, analyzing and structuring laboratory records based on information within enterprise databases, the electronic data capture (EDC) system, individual electronic laboratory notebooks (ELN) and CRO records.
A practical approach to data correction consists of splitting the problem between multiple data scientists and making use of automated data wrangling tools and scripting to facilitate data reconciliation and record verification.
Data cleaning is vital to uphold high quality data and thus accurate data decision-making. It ensures the completeness of database records (reduces instances of missing, or duplicated metadata), the consistency of the records, and their accuracy (identifies and fixes or removes incorrect metadata) across the entire study.
Serhiy: I think the best approach here is to reconcile all available metadata using prioritization and a divide-and-conquer approach. Running correlation analyses for all available collected metadata often provides deeper study insights and helps explain any observed anomalies. Often correlations can be found in secondary metadata. For example, endogenous metabolite variation may depend on collection times. Priority can be assigned to reportable data and the rest of the collected metadata can be held for cases when troubleshooting is necessary.
Elizabeth: All reportable data should be reconciled. However, each database should only reconcile metadata for which they have source documentation and report the minimum amount of metadata needed for their work. For example, clinical study sites will need to reconcile collection times since the site generates the source data. However, a bioanalytical CRO does not need collection times to analyze samples. Therefore, the CRO should not receive, report or reconcile collection times.
Lucia: Metadata is information about a dataset. It helps researchers find, understand and organize data. When cleaning data, reconciling metadata ensures consistency and accuracy across datasets, leading to high-quality data analysis. For example, standardizing biospecimens from different studies involves reconciling donor details, collection dates, sample identifiers and sample types. Other key metadata elements that should be standardized include unique IDs, date/time formats, data types, units of measurements and variable names for consistency. Using control vocabularies, consistent formats and version control ensures the metadata completeness and provenance tracking. Doing all this ensures your data is usable and consistent.
Lucia: Extensive metadata is not required for all databases, but defining key metadata such as unique identifiers, data types and the descriptions of the defined variables can make data cleaning an easier process. Deciding what metadata to include can depend on the complexity of the data and whether the data will be reused by others.
Elizabeth: No. As stated above, data cleaning is most efficient when sites, CROs and/or central labs only reconcile metadata for which they have source documentation. Minimizing unnecessary metadata across platforms greatly increases the efficiency of data cleaning. To take one example, if a bioanalytical CRO is receiving samples from a central lab and reporting data back to that central lab, the CRO should be able to report only barcode IDs and concentration data for the samples. This eliminates the need for data cleaning between the CRO and the sites.
Serhiy: There are two types of databases employed – relational data warehouses and data warehouses. For relational data warehouses, which are central stores for consolidated, highly structured information from multiple sources, their function is to support everyday operational tasks. In this instance, data cleaning will be more streamlined if only relevant and essential metadata is stored. However, in the case of data warehouses, which are designed to provide analytical insights from historical data, the approach is different.
Lucia: It is important for researchers to document actions taken to reconcile their own metadata. This documentation should include recording the metadata field name, original metadata value, revised metadata value, date of revision, the person who made the revision, the reason for the revision and any comments. Recording this information helps with tracking revisions and transparency and ensures data integrity. I often remind researchers that their future self will thank their past self for doing so.
Serhiy: The exact choice of metadata parameters to be included in the correction sheet depends on the study design and study type. If the study is exploratory in nature, the typical approach is to include all the available metadata and use automation tools, dividing the work between team members. In contrast, for confirmatory studies, priority is given to reportable metadata, filtering and identifying specific samples and recording the steps for resolving each inconsistency.
Elizabeth: Build your spreadsheet with sufficient information to identify each sample under consideration. For a clinical study, this usually means the patient ID, visit and timepoint. If available, barcode IDs are helpful for definitively identifying samples. Then state the discrepancy and the required action, including the discrepancy discovery date and the initials of the scribe. A final blank column should be included for the response of the recipient. When the recipient responds, they should also begin with the date of the response and the initials of the scribe. If three or more parties are involved in the data cleaning, for example site, central lab and CRO, add a column indicating the recipient of each query. This allows for filtering, so each party views only its own queries.
Serhiy: This depends on the study size, availability of automation tools, team experience level, type of study and set deadlines. Consistently evaluating the quality of your data is crucial for swiftly addressing any queries and preserving the reliability of your database.
Lucia: Determining the effort required to investigate each issue involves triaging them based on their impact and significance, which determines the level of effort needed to resolve them. Critical issues will have significant adverse impacts on the data quality and can include missing values or major inconsistencies. Moderate issues can include inconsistent units of measurement, mismatched data types, missing non-essential data, outdated data and partial schemas violation. Minor issues involve typos, slight variations on naming conventions, missing optional fields and extra spaces.
Elizabeth: Always do as much as possible to resolve the query. Is there a query to a CRO about a missing sample for patient 101-001? Check under patient 101-001, but also check if there are any suspicious samples for other patients from that site, or see if there are any unidentified samples for that study. Is there a query to a central lab about a missing collection time? Check the materials received with the sample and ensure the time is blank before bumping the query back to the site. Effort at each step resolves queries faster and results in less work than if queries pass back and forth with minimal investigation.
Elizabeth: Minimize, minimize, minimize! Minimizing unnecessary metadata across platforms greatly increases the efficiency of data cleaning. Also, perform updates in a timely manner and be aware of reporting deadlines in your organization. Investigate and address each round of queries before the next reconciliation transfer. This prevents duplicate queries and ensures data cleaning continues to move forwards.
Lucia: My key tip is to develop and follow a data management plan (DMP) from the start of a research project. DMPs are living documents that detail how the data will be handled during and after the research project including outlining data formats, naming conventions and metadata requirements. This proactive approach minimizes the time spent cleaning data by encouraging consistency and minimizing discrepancies.
Serhiy: Automate as much as possible, use anomaly detection tools, prioritize your work, remove redundant data values and use a divide-and-conquer approach as mentioned previously for large studies. Investing in regular data cleaning will ultimately improve the accuracy of data analysis and decision-making.
In association with: