Artificial intelligence used to optimize drug cocktail for tuberculosis
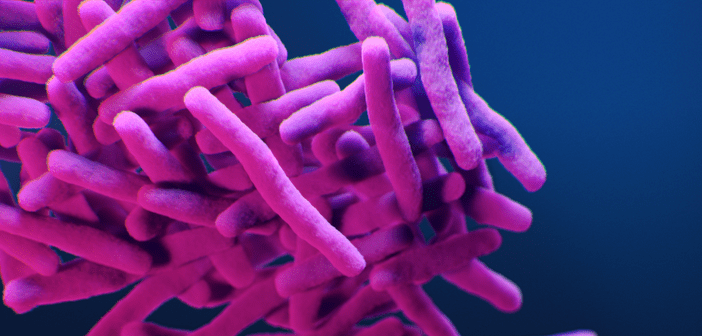
TB is the second most prolific infectious disease in the world, affecting 10 million people worldwide with a mortality rate of 1.5 million per year. The research team at the Tufts University of Medicine (MA, USA) have utilized artificial intelligence to optimize drug combinations for tuberculosis (TB) medication, thus aiming to streamline the treatment process.
Typical treatment of TB requires a trial-and-error strategy to achieve the correct three to four drugs (out of a possible 20 compounds) to create the perfect drug concoction to kill off the TB bacteria. This is particularly challenging as TB bacteria can behave very differently depending on the environment in cells and can even evolve to become resistant to antibiotics. Therefore, these drugs may need to be taken over months or years, in some cases, to eradicate the bacteria from a patient.
The research team at Tufts University has developed a set of rules which identifies the optimal drug combinations to treat patients with TB. Their findings were published in Cell Reports Medicine. This paper comes on the back of previous research whereby diagonal measurement of n-way drug interactions (DiaMOND) was used to identify high-order drug combinations. Observing how these compounds interacted allowed for more efficient treatments to be developed.
You may also be interested in:
- eBook: The role of AI in increasing efficiencies in drug discovery and development
- Perspectives on the ICH M10 guidance and its impact on the regulatory landscape for chromatographic assays
- 3D imaging solutions to support pre-clinical drug discovery
Since this research, the team has taken data from multiple large studies which investigated two-drug combinations of a possible 12 anti-TB drugs and used AI mathematical modeling which informed the set of rules developed in this study.
This new approach to the treatment process is thought to reduce the level of testing needed to discover whether a particular drug cocktail will work well together. Bree Aldridge, an Associate Professor of Molecular Biology and Microbiology at Tufts University commented:
“Using the design rules we’ve established and tested, we can substitute one drug pair for another drug pair and know with a high degree of confidence that the drug pair should work in concert with the other drug pair to kill the TB bacteria in the rodent model.”
Aldridge believes this will systemize the treatment process, as she further stated:
“The selection process we developed is both more streamlined and more accurate in predicting success than prior processes, which necessarily considered fewer combinations.”
Greater efficiency in the treatment process has the potential to have great repercussions for future treatment, as it whittles down the potential 6000 drug combinations to only those which are statistically most likely to be effective in killing the TB bacteria infection in a patient. This will majorly streamline the procedure and reduce the reliance on trial and error. This has implications not only for the future of TB treatment but also for other bacterial infections.
Sources
Larkins-Ford J, N. Degefu Y, Van N, Sokolov A, B. Aldridge B. Design principles to assemble drug combinations for effective tuberculosis therapy using interpretable pairwise drug response measurements. Cell Reports Medicine, doi: 10.1016/j.xcrm.2022.100737 (Epub, 2022), Eurekalert press release: www.eurekalert.org/news-releases/964785