Accelerating with AI: manufacturing new drugs with machine learning
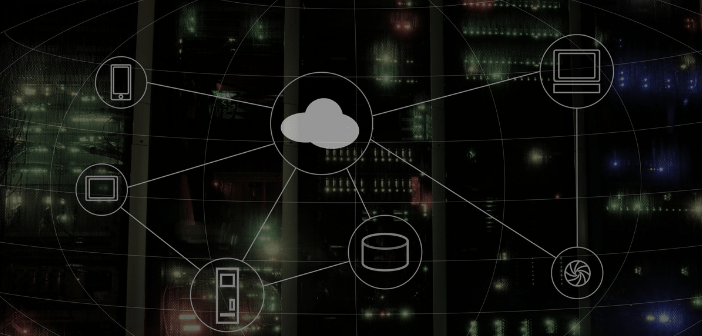
A new platform that integrates AI with automated experiments to forecast the results seen from chemical reactions has been developed, potentially expediting the development of novel drugs. Predicting the reactions of their metabolites, prior to the creation of the drug, is crucial for the drug discovery process. However, usually, this has involved a trial-and-error approach, with frequent failures in the reactions. Chemists classically rely on simulating electrons and atoms in simplified models to predict reactions, which is a rather expensive and commonly imprecise.
To tackle this issue, scientists at The University of Cambridge (Cambridge, UK) have devised a data-centric strategy derived from genomics. This method integrates automated experiments with machine learning to enhance the understanding of chemical reactivity. The research team have termed their approach chemical ‘reactome’, based on a dataset featuring over 39,000 relevant pharmaceutical reactions.
The platform is a product of a partnership between The University of Cambridge and Pfizer (NY, USA), with the results of the experiment published in the journal, Nature Chemistry.
“The reactome could change the way we think about organic chemistry,” commented the paper’s first author, Dr Emma King-Smith from Cambridge’s Cavendish Laboratory. “A deeper understanding of the chemistry could enable us to make pharmaceuticals and so many other useful products much faster. But more fundamentally, the understanding we hope to generate will be beneficial to anyone who works with molecules.”
The reactome identifies meaningful relationships among reactants, reagents and reaction performance within the data, whilst also highlighting deficiencies in the data itself.
“High-throughput chemistry has been a game-changer, but we believed there was a way to uncover a deeper understanding of chemical reactions than what can be observed from the initial results of a high-throughput experiment,” explained King-Smith.
“Our approach uncovers the hidden relationships between reaction components and outcomes,” added Dr Alpha Lee, who led the research. “The dataset we trained the model on is massive—it will help bring the process of chemical discovery from trial-and-error to the age of big data.”
In a linked study published in Nature Communications, the same research team created a machine learning technique to aid chemists in implementing precise changes to predefined regions of molecules, to expedite the drug design process. The method allows chemists to modify complex molecules, such as a last-minute design change, without the need to construct them anew from the beginning. Their machine learning model can also predict where a molecule would react, and how the site of reaction can differ as a function of the various reaction conditions.
“The application of machine learning to chemistry is often throttled by the problem that the amount of data is small compared to the vastness of chemical space,” commented Lee. “Our approach—designing models that learn from large datasets that are similar but not the same as the problem we are trying to solve—resolve this fundamental low-data challenge and could unlock advances beyond late-stage functionalization.”
Sources: King-Smith E, Berritt S, Bernier L et al. Probing the chemical ‘reactome’ with high-throughput experimentation data. Nat. Chem. doi:10.1038/s41557-023-01393-w (2024) (Epub ahead of print); https://phys.org/news/2024-01-drugs-machine.html