Data acquisition modes for LC–MS-based untargeted metabolomics
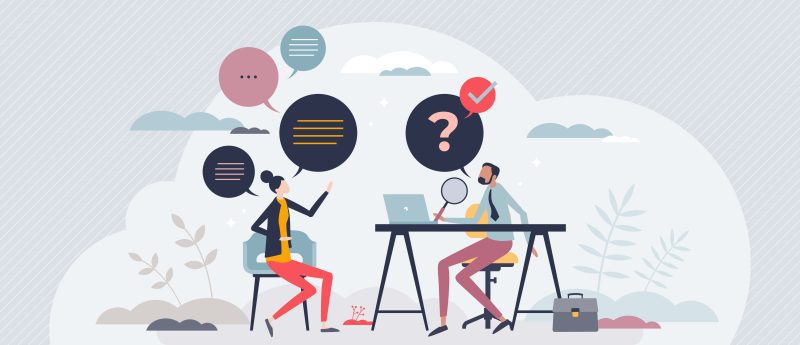
In this interview we caught up with Jian Guo (University of British Columbia, Vancouver, Canada) to discuss his presentation at ASMS 2020 Reboot. Jian discusses his research inspirations, current focus and what inspired his presentation exploring which data acquisition modes to use for LC–MS-based untargeted metabolomics.
Make sure you don’t miss Jian’s presentation, ‘Comparison of three common data acquisition modes in liquid chromatography – mass spectrometry,’ taking place online on Thursday 4 June from 09:10 [CDT] at ASMS 2020 Reboot.
My name is Jian Guo, a second year PhD student from the Huan Lab in the Department of Chemistry, at the University of British Columbia in Canada. My research involves mass spectrometry as a prominent analytical tool for the unbiased measurement of metabolites in biological samples. For the past 1 year and half, I have been invigorated and inspired to work on the establishment of multiple analytical workflows for a plethora of biological applications using liquid chromatography–mass spectrometry (LC–MS). I have developed untargeted LC–MS methods for global metabolomic profiling of serum, urine, microbial media, tissues, cancer and immune cells for diverse independent and collaborative studies. One of the collaborative works has been completed and published in ACS Infectious Diseases [1]. Another collaborative work has also been published in Frontiers in Immunology [2]. In addition to my biological application related projects, I have also independently conducted fundamental studies on LC–MS data acquisition optimization to cover more metabolic molecules with confidently positive identification. I compared three developed LC–MS data acquisition methods and determined the optimal for given untargeted metabolomics projects. This work has been published in Analytical Chemistry [3].
QWhy is metabolomics an important area of bioanalysis?
Metabolomics is a systematic study of the entire set of metabolites in tissues, biofluids and cells. It is important for bioanalysis because many diseases are attributed to metabolic disorder. In order to discover the biomarkers for certain diseases, metabolomics is essential. Besides. it also fills the gap between genome, proteome and phenotype. A comprehensive network about how biomolecules interact with each other in the biological system can be mapped with metabolomics added into the omics studies. For example, some bioactive metabolites have been found responsible for the modifications on genes, proteins and lipids to regulate the biological system. Metabolomics can guide researchers, allowing them to see the regulated pathways more clearly [4]. However, metabolic changes occur rapidly and metabolomics technologies are highly warranted to obtain the accurate and precise information about the metabolome and its alterations in different biological conditions. Metabolomics has emerged as a powerful technology that has been widely used in many research areas, including but not limited to, the discovery of disease biomarkers, study of metabolic pathways, and searching for bioactive chemicals that modulate disease phenotypes, such as leukemia, diabetes et cetera. More endeavor should be put into metabolomics development in the future.
QCan you please summarize the three data acquisition modes you will be discussing in your presentation?
Full-scan, data-dependent acquisition (DDA) and data-independent acquisition (DIA) are the three most commonly used data acquisition modes in LC–MS-based untargeted metabolomics, with each having its own advantages and limitations. To the best of our knowledge, most researchers select the mode already established in their lab and the literature of choosing an appropriate acquisition mode for given untargeted metabolomics projects is still lacking. It is an important yet underrated research topic: which acquisition mode is more suitable for a given untargeted metabolomics application? The conventional approach, full-scan followed by structure identification, has been well established and highlighted by its capability to detect the largest number of metabolic features and provide the highest quantification precision, but it requires extra instrumental analysis time and sample volumes. DDA is convenient to use, as it streamlines metabolic profiling and metabolite identification with high-quality MS2 spectra, although it performs deficiently in the detection of low-abundance metabolites and its quantitative precision is not as good. DIA is capable of identifying low-abundance metabolites, but the deconvolution complexity rises greatly. However, it is believed that DIA will be more useful in the future when a better deconvolution algorithm is developed.
You might also like…
QWhat do you hope attendees will gain from your presentation?
From this presentation, attendees will gain an in-depth understanding of how each data acquisition mode works in a great amount of detail. Understanding the mechanism helps them decide if the acquisition mode they are currently using should be changed or fine-tuned to better fit their research needs. The attendees will be able to learn the pros and cons of full-scan, DDA, and DIA modes in terms of their metabolic features’ coverage, quantitative precision, MS2 spectra number, MS2 spectra quality and convenience in different scenarios. I hope it can provide some take-home messages which will help attendees choose the best data acquisition mode for their metabolomics projects.
QWhat impact do you think the shift to an online format will have on ASMS 2020?
There will be much less opportunity for attendees to have face-to-face discussions with the speakers. It will definitely hinder us from knowing more people as the online format is less convenient for personal interactions. But, I think it is better than not having it at all. We can still watch the videos and appreciate the valuable content from thousands of speakers and posters.
[1] Tang X, Kudo Y, Guo J et al. Cariogenic Streptococcus mutans produces tetramic acid strain-specific antibiotics that impair commensal colonization. ACS Infect. Dis. 6(4), 563–571 (2020).
[2] Dong Y, Arif A, Guo J et al. CD44 loss disrupts lung lipid surfactant homeostasis and exacerbates oxidized lipid-induced lung inflammation. Font. Immunol. 11(29) (2020).
[3] Guo J and Huan T. Comparison of full-scan, data-dependent and data-independent acquisition modes in liquid chromatography-mass spectrometry based untargeted metabolomics. Anal. Chem. doi:10.1021/acs.analchem.9b05135 (2020)(Epub ahead of print)
[4] Rinschen MM, Ivanisevic J, Giera M and Siuzdak G. Identification of bioactive metabolites using activity metabolomics. Nature. 20, 353–367 (2019).
The opinions expressed in this feature are those of the author and do not necessarily reflect the views of Bioanalysis Zone or Future Science Group.
Our expert opinion collection provides you with in-depth articles written by authors from across the field of bioanalysis. Our expert opinions are perfect for those wanting a comprehensive, written review of a topic or looking for perspective pieces from our regular contributors.
See an article that catches your eye? Read any of our articles below for free.