Asthma biomarker detected by nasal brush test
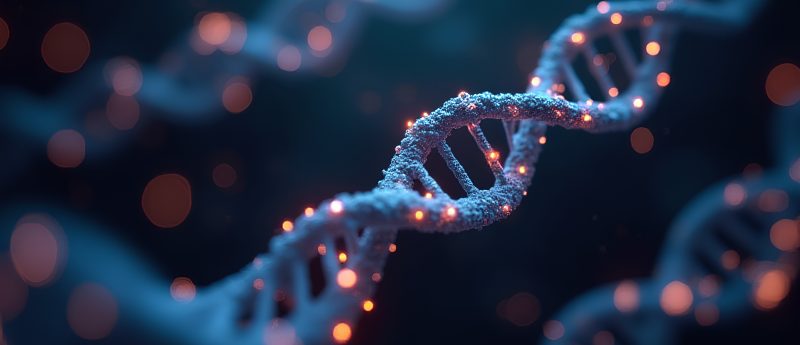
A team from the Icahn School of Medicine at Mount Sinai (NY, USA) have created a diagnostic test that could identify a biomarker for asthma, differentiating it from other respiratory conditions. The research, published in Scientific Reports, details how the nasal brush and subsequent analysis of the asthma biomarker could provide binary results definitive of the disease.
Supinda Bunyavanich, researcher at the Icahn School of Medicine, commented: “Mild to moderate asthma can be difficult to diagnose because symptoms change over time and can be complicated by other respiratory conditions. Our nasal brush test takes seconds to collect – for time-strapped clinicians, particularly primary care providers at the frontlines of asthma diagnosis, this could greatly improve patient outcomes through early and accurate diagnosis.”
Researchers utilized the nasal brush to acquire genetic data from patients, which was applied to machine learning algorithms. The machine learning analysis identified a 90-gene biomarker indicative of asthma.
Gaurav Pandey, another researcher at the Icahn School of Medicine, added: “One of the most exciting components of this study is demonstrating the power of machine learning when applied to biomedical data. Collaborations between computational scientists and biomedical researchers and clinicians are advancing medicine at an inspiring pace – we have the power of insights we didn’t have many of in the past and that opens a window to an entirely new world of diagnostic tools and treatments.”
The team hopes to conduct a study on a larger population of patients, enabling it to be brought into clinical practice. Bunyavanich concluded: “With prospective validation in large cohorts, our asthma biomarker could lead to the development of a minimally invasive test to aid asthma diagnosis at clinical frontlines where time and resources often preclude pulmonary function testing.”
Sources: Pandey G, Pandey OP, Rogers AJ et al. A nasal brush-based classifier of asthma identified by machine learning analysis of nasal RNA sequence data. Sci. Rep. doi:10.1101/145771 (2018); www.sciencedaily.com/releases/2018/06/180611133429.htm