2013 Young Investigator Award Finalist: Andris Jankevics
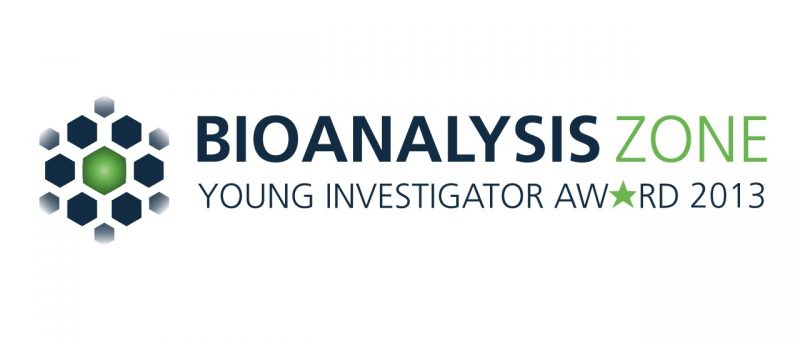
Nominee:
Nominated By:
Supporting Comments:
What drove you to choose a career in bioanalysis?
During my undergraduate studies in Chemistry, I developed a strong interest in subjects related to modern bioanalytical methods and their applications. These methods generate huge amounts of data, and my increased interest in bioinformatics accelerated my involvement with projects requiring computational support. My knowledge of the physico-chemical factors underlying various analytical methods (mostly NMR and LC–MS) and my understanding of modern statistical methods (I obtained an MSc in Mathematics) not only was helpful for understanding the complex nature of bioanalytical data, but also was useful in communicating with my collaborators during the development of powerful new approaches to data processing.
Describe the main highlights of your bioanalytical research, and its importance to the bioanalytical community both now and in the future
My PhD research was related to the high-throughput analysis of the metabolome using modern high-resolution MS equipment. Typical experiments in metabolomics produce large amounts of data, and the rapid and reliable computational analysis of such datasetsis an important challenge. Together with our biologist partners we developed open source software for the analysis of metabolomics data. A highly modular design of the software allowed us not only to fine-tune the analytical components relevant for a current study design, but also to share intermediate analysis results with the biologists and analytical chemists on the project in an intuitive way. This new approach facilitated the communication between researchers with different backgrounds and resulted in the rapid discovery of bottlenecks in experimental design, bioanalytical methodology or data analysis. Very often new analytical approaches were suggested by our collaborators, who for the first time had full access to their data, in contrast to previous software tools where users could interact only with the final result or had to switch between several different software packages). In the last 2 years, I co-organized four hands-on workshops teaching new users how to exploit our new tools to make best use of their metabolomics datasets.
Describe the most difficult challenge you have encountered in the laboratory and how you overcame it?
At the beginning of my PhD project I started to work on existing software developed in our group. These tools had a unique design and provided some powerful functions for data processing not available in similar software packages. But sometimes a closer look at the results showed problems: for example, the algorithm used to detect a signals from raw measurements data set was not performing as good as in other software packages. This posed a major challenge: how to improve our software, without having to reinvent the wheel? I set myself the goal to make the processing pipeline far more modular, so that researchers could combine existing functionality from different software packages in a single data processing framework. I achieved this by integrating our previous tools with the statistical programming language R. As a result, users can now analyze their data with the most up-to-date LC–MS analysis algorithms at each step in the pipeline and utilize the very powerful statistical and visualization functionalities of R. Using the easy-to-learn R language as the basis for my developments makes the results of my work accessible to a much wider range of researchers, who, in turn, can contribute new ideas and improvements.
Where do you see your career in bioanalysis taking you?
I am currently continuing to develop and improve LC–MS data processing algorithms, having moved to a postdoctoral position at the University of Manchester. Although the approaches developed in my PhD work led to a fuller understanding of the metabolome, there is a still lot of room for improvement.
I am now focusing on two main areas:
- Making the data processing and data interpretation more accessible to non-bioinformaticians, in particular by developing (semi-)automated tools for data processing and user-friendly interfaces
- Developing automated tools to collect and summarize published metabolomics data in an integrated way. Huge amounts of information are currently becoming available, but are underexploited due to the computational challenge of bringing data from very diverse sources together.
My career in bioanalytics will hopefully allow me to be actively involved in providing expertise and tools to link together collaborators with a wide range of background knowledge help us to formulate exciting biological questions and develop the best bioanalytical strategies to answer them.
How do you envisage the field of bioanalysis evolving in the future?
The improvement in technology in the near future will undoubtedly lead to a new generation of mass spectrometers with even higher resolving power and mass accuracy – and with a further explosion of the amount of data generated. I expect that the development of analytical platforms will have to go hand-in-hand with the evolution of the available software tools and that as a result we will achieve a significantly increased coverage of the metabolome in untargeted experiments. Metabolic profiles, which are close representatives of an organism’s phenotype, will increasingly be combined with other global molecular profiles, such as genomic, transcriptomic and proteomic data to provide an unprecedented insight into the function of metabolic systems. New powerful and comprehensive bioinformatics techniques will facilitate the adoption of untargeted metabolomics not only as a tool for biological hypothesis generation, but will open up entirely new areas, including applications in rapid disease diagnostics, the development of new drugs targeting metabolism and metabolomics-informed personalized medicine, based on comprehensive time-resolved metabolite profiles for individual patients.
Please list 5 of your recent publications, and select one that best highlights your career to date in the field of bioanalysis.
This publication highlights the combination of new computational approaches, and a novel experimental design for the metabolomics analysis of an extremely large number of analytical replicates:
- Jankevics A*, Merlo ME*, de Vries M, Vonk RJ, Takano E, Breitling R.Metabolomic analysis of a synthetic metabolic switch in Streptomyces coelicolor A3(2). Proteomics 11(24), 4622–4631 (2011). (*These authors contributed equally).
The following three publications demonstrate new computational tools to solve major bioanalytical challenges in metabolomics:
- Scheltema RA*, Jankevics A*, Jansen RC, Swertz MA, Breitling R.PeakML/mzMatch: A file format, java library, R library, and tool-chain for massspectrometry data analysis. Anal. Chem. 83(7), 2786–2793 (2011). (*These authors contributed equally).
- Jankevics A, Merlo ME, de Vries M, Vonk RJ, Takano E, Breitling R. Separatingthe wheat from the chaff: A prioritisation pipeline for the analysis of metabolomicsdatasets. Metabolomics 8 (Supp. 1), 29–36 (2011).
- Chokkathukalam A*, Jankevics A*, Creek DJ, Achcar F, Barrett MP, Breitling R. mzMatch-ISO: an R tool for the annotation and relative quantification of isotope-labelled mass spectrometry data. Bioinformatics 29(2), 281–283 (2011) (*These authors contributed equally).
Finally, this review paper summarizes developments in experimental design and data handling for large-scale metabolomics studies and outlines future perspectives for further research in metabolomics:
- Merlo ME, Jankevics A, Takano E, Breitling R. Exploring the metabolic state of microorganisms using metabolomics. Bioanalysis 3(21), 2443–2458 (2011).